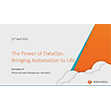
Is Bad Data Costing You Millions?
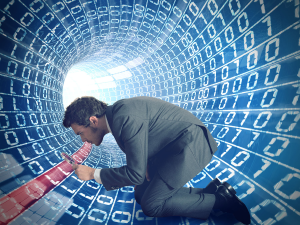
It’s no secret that harnessing the power of big data has the potential to be a huge boon for enterprises. Scores of analyst reports offer the promised land of predictive analytics and countless other benefits, making the rush to jump on the big data bandwagon seem like a no brainer for CIOs. Unfortunately, many companies often begin down the path towards big data without a solid understanding of the keys to success.
One critical characteristic of big data that is often overlooked is the prevalence of bad data. Despite its sinister sounding name, bad data isn’t inherently evil. Rather, at the crux of the bad data issue is poor or inconsistent data quality. Gartner estimates that poor data quality costs the average business $13.5 million each year. Unbeknownst to most, a third of data scientists along with other data and business intelligence experts spend 90 percent of their time “cleaning” raw data. In a nutshell, bad data is bad for business.
Bad Data – How it happens
No organization is immune to bad data. Most often, bad data arises from technological limitations and poor data management planning. Perhaps a company has poor data standardization practices. Maybe someone’s manual spreadsheet has excessive fields that lack data value definitions. Or quite possibly it’s something as simple as a duplicate record or typo. Adding to the bad data issue is the fact that today’s data management systems and solutions didn’t exist 15, 10 or even five years ago. With technology advancing faster than businesses ability to keep up, enterprises are faced with increasingly complex IT infrastructures that are handicapped by legacy systems.
In real-world terms, bad data comes in all shapes and sizes. In the case of managing software inventory, there are enormous cost, productivity and security implications resulting from incomplete data about software
applications installed across an enterprise. For instance, it’s common to run multiple versions of the same software application across different users and servers in an organization. However, once these multi-version applications reach a critical maximum – typically three versions or more – the result is uncontrolled IT maintenance expenditures and the potential for unforeseen security risks.
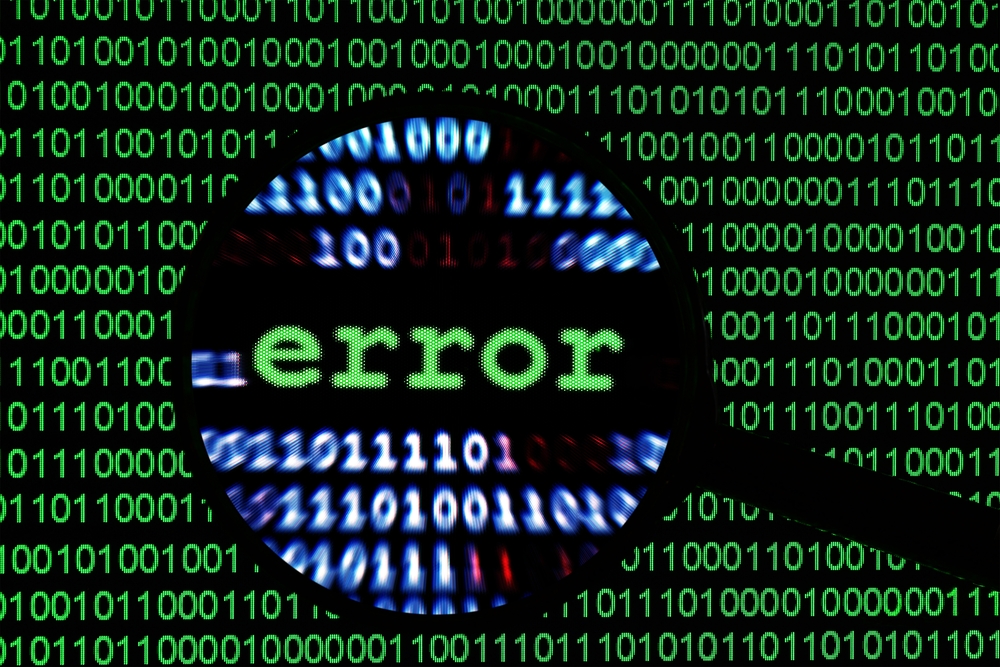
(image courtesy alexskopje/Shutterstock.com)
Bad data also threatens IT’s ability to accurately measure service level agreements (SLAs). Managing internal SLAs is challenging enough; couple that with the SLAs of multiple Managed Services Providers (MSPs), each of whom work within a different segment of the enterprise’s overall environment, and you have a genuine challenge on your hands. Since each discipline often has its own defined process to meet individual SLAs, the resulting silos cause a breakdown in confidence in the accuracy and validity of the SLA measurements. In addition, because IT must rely on MSPs to self-report SLA measurements, enterprises are subject to an added level of vulnerability and economic uncertainty.
So what can enterprises do to reign in this rogue information?
Getting Quality Data
Quality data collection is the key to big data success and the ability to perform intelligent data analytics. For most, collecting quality data is governed by the three Vs: Velocity – how quickly and often information is collected; Volume – how much data is collected; and Variety – the different types of data collected. However, there is a fourth – often neglected, but more important – V, Validation. It doesn’t matter how fast, how much or what type of data an enterprise collects. If the data is inaccurate, it is useless for analysis.
To perform intelligent data analysis, enterprises need a solution that:
- Delivers immediate insight to the enterprise’s current state
- Has real-time data—as well as a complete history—about people, processes and products
- Sources all relevant IT data for visibility across the entire enterprise
- Builds the foundation for predictive analytics and prescriptive modeling
- Deploys quickly and automatically, providing fast ROI
- Adapts to data volume, velocity and variety, while providing data validation
The business implications of poor IT data quality are immense and extend throughout all levels and components of the enterprise: the inability to accurately measure, validate or control the financial performance or cost-effectiveness of IT, the risk of expensive IT outages, lagging productivity, inefficiency and duplicated efforts, lost business opportunities, and dampened competitiveness. This goes beyond technical headaches and missed revenue opportunities, as poor data quality poses risks to consumers and the U.S. economy – which is estimated to lose $3 trillion per year due to bad data.
This is today’s biggest risk, and biggest opportunity. To make sound, strategic IT decisions, companies need data that is complete, up-to-date and accurate. By implementing an effective data collection and purification process, enterprises can empower themselves with the knowledge to successfully assess data quality, and implement the right tools and rules to improve the accuracy of their data and the associated decisions based on that data. Remember that it’s not just about capturing and managing big data, but also validating this information in order to create a dependable, quality data set that can then be used for effective analytics.
About the author: As CEO, Gary Oliver is responsible for leading the strategic direction and execution at Blazent. He has over 25 years of experience in both IT executive roles and in leading high growth IT software organizations.
Related Items:
Five Steps to Fix the Data Feedback Loop and Rescue Analysis from ‘Bad’ Data
(feature image courtesy alphaspirit/Shutterstock.com)