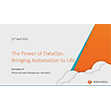
Is Your Organization Making the Best Use of Its Big Data?
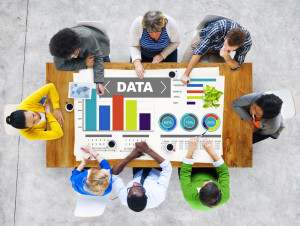
(Rawpixel.com/Shutterstock.com)
The term big data was originally coined to describe data whose size, variety and structure could not be stored, managed or processed via traditional database technologies. Over the past decade however, the scope of the term has grown dramatically to represent not only data but also the associated hardware, software and services.
Big data technologies have evolved significantly over the past few years. Data processing, which previously was a passive activity, now happens in real time, resulting in continuous access and easier analysis of data at a large scale. The result? Superior datafication for businesses – they now have the ability to discover previously unknown trends and relationships using data. With the advent of the connected eco-system and the birth of the Internet of Things (IoT), all of these new systems and devices have multiplied the scale and scope of data exponentially. This has also led to the birth of new processes and policies that have enhanced the speed and efficiency at which data is captured, managed and analyzed today.
What Are the White Spaces in The Current Big Data Landscape?
Despite the abundance of big data technologies available in the market today, enterprises struggle to take advantage of big data, because they fail to fulfill the following requirements:
- Implementing mechanisms to efficiently consolidate data from a large number and variety of sources
- Effectively industrializing the entire data life-cycle
- Consolidating technology stacks to successfully facilitate effective aggregation, ingestion, analysis and consumption of data to provide value and ROI from big data implementations
Enterprises must jump over quite a few hurdles in order to implement productive and efficient big data strategies.
What Steps Should an Enterprise Take to Successfully Implement Big Data?
In order to tap into the humongous potential that big data has to offer, enterprises should make sure to take the following steps:
- Define: Codifying a precise problem that can be solved using data.
- Identify: Experts within the enterprise need to agree upon what type of data should be collected, and what sources to collect data from, and the way it should be collected.
- Model:Creating the right data model is extremely important – it forms the core of the implementation by processing the collected data. Patience is also key when creating data models. Enterprises often move forward and increase the data sample size without taking the time to verify whether a model is correct or not. Once a data model has been tested and is successful, enterprises still need to be careful, though. The data sample size should be increased gradually. A strong assurance strategy that filters out bad data and ensures data quality needs also needs to be setup during this phase.
- Implement:Enterprises need to make sure to choose the right technology stack when industrializing, aggregating, ingesting, processing and consuming data. This is where a strong platform assurance strategy needs to be incorporated.
- Optimization via Assurance:Last but not least, even after implementation there needs to be constant monitoring of the data model to ensure best possible results are gained. This may involve recreating models and re-implementing to ensure optimal calibration of the data model and the technology platform used to process and consume the data.
(THesIMPLIFY/Shutterstock.com)
What Does an Effective Big Data Assurance Strategy Encompass?
Building a cohesive big data strategy allows enterprises to spend less time worrying about their technology and more time focusing on creating value via measurable and repeatable methodologies. Teams now have more time to focus on technical challenges, such as categorizing and identifying associated key activities in the data life- cycle. However enterprises should not forget to also validate and verify these activities to ensure they can maximize value creation from its big data implementations, right from ingestion to the consumption stage. The key elements of a holistic assurance strategy include:
- Data Quality Assurance: When worrying about data quality assurance, it’s important to keep correctness, completeness, and timeliness of the data collected in mind. By screening the data at the source itself to ensure correctness and completeness, enterprises can ensure that it is correct, complete, and timely. Upstream as well as downstream quality assurance is also a must for businesses, and can be capitalized on through standardized and automated, self- service assurance platforms.
- Platform Assurance: Platform assurance not only is data quality critical, but it is also important to assure the functional as well as non-functional (such as performance) parameters of the platform. This is done by testing algorithms that are written to cleanse, process and transform the data along with the technologies used to ingest, process and consume data. It’s also imperative to predefine a set of quality metrics which should be continuously scrutinized via dashboards and reports. This will ensure that the platform performs its allotted tasks at the highest level, at all times.
To summarize, big data today is much more than a buzz word and the benefits that can be reaped from datafication are real and tangible. However, realizing the value from the big data is not as simple to master. It requires its due share of respect in the form of due diligence. Unfortunately, most organizations fail in their big data projects due a number of reasons: such as not setting a defined problem statement, spending the required time to create a robust data model, or, setting up a holistic data assurance strategy that would enable organization to address both the above oversights as early as possible. Due to these lapses, organizations often face disappointment as they are unable to leverage the value from their data.
About the author: Bharath Hemachandran heads Wipro’s Big Data Assurance Practice. With over a decade of experience, Bharath is focused on deciphering big data and working towards innovative uses of artificial intelligence and machine learning in quality assurance. Bharath has worked in a variety of technical and management positions in companies throughout the world.
Related Items:
What Data Science Skills Employers Want Now
Is Bad Data Costing You Millions?
November 22, 2024
- DataOps.live Achieves SOC 2 Type II Compliance
- LogicMonitor Gains $800M in Strategic Investment to Scale Global Operations
November 21, 2024
- Snowflake Agrees to Acquire Open Data Integration Platform, Datavolo
- Denodo Platform 9.1 Brings New Advanced AI Capabilities and Enhanced Data Lakehouse Performance
- Teradata AI Unlimited in Microsoft Fabric Public Preview Now Available Through Microsoft Fabric Workload Hub
- Zilliz Cloud Powers GenAI Readiness with Cost-Effective Enterprise-Grade Performance and Scalability
- Snowflake and Anthropic Team Up to Bring Claude Models Directly to the AI Data Cloud
- Duality AI Launches EDU Subscription to Empower Aspiring AI Developers with Digital Twin Simulation and Synthetic Data Skills
- Striim Offers Mirroring Solution for SQL Server to Fabric at Microsoft Ignite
November 20, 2024
- Anaconda Unites Teams Across Data Skill Levels With Anaconda Toolbox for Excel
- StarTree Unveils Innovations to Tackle Real-Time Data Scaling Challenges
- Introducing Crunchy Data Warehouse, a Modern Postgres Analytics Platform
- Zettar Advances Data Movement in Collaboration with MiTAC Computing and NVIDIA
- Matillion Leverages Simbian’s AI to Streamline Security and Boost Efficiency
- CData Launches Free Connect Spreadsheets Product to Simplify Access to Enterprise Data for Excel and Google Sheets Users
- Graphwise Introduces GraphDB 10.8 with Multi-Method RAG for GenAI Applications