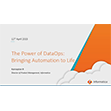
Harnessing Predictive Analytics for Infrastructure Monitoring
With the radical transformation of the datacenter by the arrival and rapid adoption of virtualization and the cloud, the added complexity requires a new approach to monitoring this environment.
The ability to dynamically assign or reduce computing power, and that too with the luxury of distributed, heterogeneous work units renders traditional IT monitoring tools useless.
At a leading US university, even while reducing the number of physical servers from 1,000 to 100, the number of virtual machines increased to 7,000 – trying to track which application lived on which virtual machines on which physical server itself was a challenge, let alone trying to correlate the impact of one virtual machine on another. The sprawling, distributed IT components demand intelligent ways to manage and monitor this infrastructure.
This pain has been the main driver for the application of analytics in the field of IT monitoring. The IT customer’s demands have always been dynamic, and IT departments have traditionally reacted by provisioning for the peak demand, resulting in wasted idle compute resources.
Even the usage of application resources is dynamic by the hour and day of week, and it is increasingly important for IT departments to understand the behavior pattern of their network and applications in addition to the computing resources. The number of users, the response times, the queued messages, the database query rate – all vary by time of day and understanding the usage pattern and correlation between them helps isolate the root cause of IT service problems.
There are several components to these solutions – the first is collection of data from the IT infrastructure, and in large enterprises with tens of thousands of nodes, this can result in millions of data points every few minutes. You need to be able to collect, process, and store these metrics in close to real time since there are no “idle” periods which would allow batch processing. Some solutions use distributed processing and databases to address this volume of data. Big data methods are also possible.
The analytics engine then processes this data to generate baselines and behavior analysis to determine trends based on time of day and day of week. This analysis feeds into a workflow engine to allow automation for provisioning and de-provisioning of resources depending on the projected requirements. This time based analysis fits well into the elastic nature of the cloud platform allowing for efficient use of resources.
A correlation engine needs to be able to correlate the behavior analysis for the different metrics and derive useful service or application patterns based on this data. Obvious correlation models such as high user counts with high database transactions allow detecting problems when both these patterns diverge from their correlated behavior patterns. However, this area of data analytics is still very complex and nascent at this stage.
Automation and analytics are smart product features focusing on reducing the administrative burden in today’s distributed cloud environments. Keeping business necessities in mind, these innovative features are pragmatically relevant and a must in today’s IT networks.
Rajib Rashid is CTO of Zyrion Inc., a leading provider of Cloud and Application Performance Monitoring software for large to mid enterprises and Service Providers. He is an active Internet pioneer and has many years of experience in engineering large ISP networks such as Verio and NTT. You can read more about his current company and their monitoring solution here.