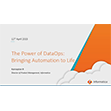
Breaching the Big Data Barrier in Healthcare
The financial sector has woven big data analysis seamlessly into its operation. Scientific research has benefited from an open source aspect of sharing big data to the cloud, helping to prompt the discovery of the Higgs Boson.
Yet for some reason, the healthcare industry falls behind its financial and scientific counterparts so much that modern medicine seems to be stuck behind a wall.
If medicine is waiting behind the big data barrier, what materials were used to construct that wall? What is the best way to breach it? To answer these questions and more, Forbes brought together a panel of medical experts from across the industry. Glen de Vries, co-founder and president of Medidata, moderated the panel consisting of Harlan Krumholz, Professor of Medicine, Epidemiology and Public Health at Yale University; Andy Slavitt, Group Executive Vice President at Optum; and Peter Tippett, Chief Medical Officer of Verizon.
To be fair, it is not as if big data has not had its effect in medicine. According to Krumholz, 90% of people who come into an ER are treated within 90 minutes. A few years ago, approximately half of all patients had to wait at least two hours—an eternity for high-risk heart attack patients. As a result, mortality rates from heart attacks have dropped 25% over the last few years.
Further, the methods that credit card companies are using for fraud detection can be adapted to suit healthcare. According to Tippett, fraud contributes $70 billion annually to the cost of government programs like Medicare and Medicaid. Tippett’s company, Verizon, has even done some work in the area, compiling a list of “naughty and nice” IP addresses that gets updated every four minutes.
However, those two examples barely scratch the surface of what big data can do for healthcare. In his book, Physics of the Future, and in his corresponding keynote speech at Supercomputing, Michio Kaku predicts a not-too-distant future (by the year 2040) where most diagnoses and consultations are computerized and instantaneous. Something is holding healthcare back from that shiny future.
“Trust is the core issue,” said Tippett. The panelists disagreed on a few specific issues (quality control being one, but more on that later), but the general consensus seems to be that there exists a general, overarching lack of trust, from patients to doctors to executives.
Following the mandate that all medical records must be digitalized, there has been a tidal wave of healthcare data out there waiting to be aggregated and utilized by someone. This is the case in theory, anyway. In practice, a great deal of that data may not actually be all that useful.
According to Krumholz and Slavitt, the vast majority of collected data over the last 17 years is of the drug utilization and administrative variety. That information can help improve efficiency, such as the aforementioned example of ER delay time decreasing over the last few years, but does little in advancing personalized medicine.
The drug information gets us a little closer but even that impact is limited. According to Krumholz, a company will gladly run 30-odd trials of a pill like Lipitor in order to improve and customize it. However, not enough credible comparative studies are done to compare the effects of Lipitor to similar pharmaceuticals on the market.
Either way, that sort of information does not particularly lend itself to solving questions like, ‘when will that man suffer his next heart attack?’ that the constant collection of data from monitoring systems would. “If we use that (drug and administrative) data to solve problems it shouldn’t solve,” said Slavitt, “we’re doing everyone a disservice.”
One problem is that collecting all of that monitor data is a huge undertaking for even one hospital. As explained in this article, the amount of points in the dataset could regularly reach the trillions for a place like the Children’s Hospital of Los Angeles. But that, in essence, is the challenge of big data: finding insights from mega datasets. Genomics, a field which hopes to inform the healthcare industry soon, is at the point where sequencing a person’s DNA takes a matter of hours and a few thousand dollars. A decade ago, that same process took several years and a few billion dollars.
The problem lies in availability, not in volume. Because companies are at a loss on how to gain that useful information or how to properly use it, they revert back to gut-driven instead of data-driven diagnoses.
“Companies that are focused on an outcome that you need data to get at are more successful,” said Slavitt. “For the rest of us, because we don’t have access to data, we stop asking data-driven questions.” With regard to data in medicine, there seems to be a general sense of “how do we deal with this in a legal and efficient way?”
Since companies are slow to realize exactly how they plan to use an individual’s sensor information, the individual is less inclined to allow their data to be used in scientific studies. But if those people have a succinct explanation for a) how their data will be used and b) to what extent it will directly impact the individual in question, the stigma against sharing their information would disappear.
“If you put control of their data in their own hands,” said Tippett, “the vast majority of people will say, ‘of course you can use it for research.’” Indeed, we highlighted a case a couple of weeks ago where a computer-savvy Italian professor named Salvatore Iaconesi grew frustrated with the fact that his doctors were making decisions based on gut and instinct instead of the swath of opinions and information that could have been available. As such, Iaconesi requested his digital medical file and shared it on an open source forum to arrive at a diagnosis and a treatment plan.
Iaconesi controlled his data, knew exactly what has happening with it, put it on an open forum, and received terrific medical advice.
This is great, but there is one final problem with that scenario: it is much less likely to happen in the intense for-profit nature of medicine in the United States. People are wary of their insurance companies denying coverage as a result of ‘pre-existing conditions.’ That wariness is understandable if insurance companies are able to deny or hike up coverage based on what they learn from the collection of big data.
When healthcare becomes about profit, quality suffers, according to Krumholz in one of the more entertaining exchanges in the panel:
“You can save money by cutting corners on quality,” said Krumholz.
“That’s not what happened in the auto industry, the Japanese took over because they had better quality,” said Tippett.
Krumholz responded by saying, “Yeah but the Japanese aren’t going to take over Presbyterian.” The Japanese can export their cars to America but they cannot well export their hospitals.
There are problems to solve before big data can really infiltrate the healthcare industry and do what it has done for the financial sector. Some of that has to do with the for-profit nature of medicine in the States, but a larger portion deal with the trust issues the public harbors toward the industry. Until those are solved, healthcare will stay stuck behind the big data barrier.
Related Articles
Hospitals Take to Mining for Lives
Can Hype Spell Hope for Predictive Healthcare Analytics?