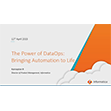
How Baidu Uses Deep Learning to Drive Success on the Web
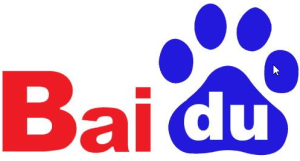
The Chinese Web giant Baidu is investing heavily in deep learning technologies as it seeks to drive intelligence from big data using high performance computing (HPC). From speech and facial recognition to language transaction and Web search, Baidu relies on deep learning and artificial intelligence technologies to improve a range of customer-facing applications.
Dr. Ren Wu, a distinguished scientist at Baidu’s Deep Learning Institute (IDL), discussed Baidu’s use of deep learning technologies in a keynote presentation at Tabor Communication’s recent Enterprise HPC event in Carlsbad, California. In his keynote, titled “HPC – Turning Big Data Into Deep Insight,” Wu explains how Baidu was an early adopter of deep learning technology and has continued to aggressively develop its expertise in the field in reaction to its early success.
The commitment to deep learning comes from the top, namely Robin Li, the co-founder, CEO, and chairman of Baidu and the president of its IDL subsidiary. That executive-level support has resulted deep learning technologies becoming pervasive across Baidu. “We apply deep learning technology to every single application, and every single one has observed tremendous performance enhancements,” Wu told the audience.
As Wu sees it, deep learning is critical for making sense of the mountain of data that’s being generated on the Internet. The company, which has been called “the Chinese Google” (although Wu says Google is actually “the American Baidu”), stores 2,000 PB of data, and processes anywhere from 10 to 100 PB of data a day, according to Wu’s presentation. It indexes upward of 1 trillion pages, which are updated at the rate of 1 billion to 10 billion per day.
The combination of big data and advances in HPC have enabled the field of deep learning to progress in ways that nobody had predicted, Wu said. “First it’s the Internet and the data explosion. We have much more data and labeled data, so we can now show our neural networks much more examples, so that a network can learn so many different things” that were previously not possible, he said. “That’s one side of the story. What I think is even more important is we have a lot more computational power in our hands. That computational power is the real driving force.”
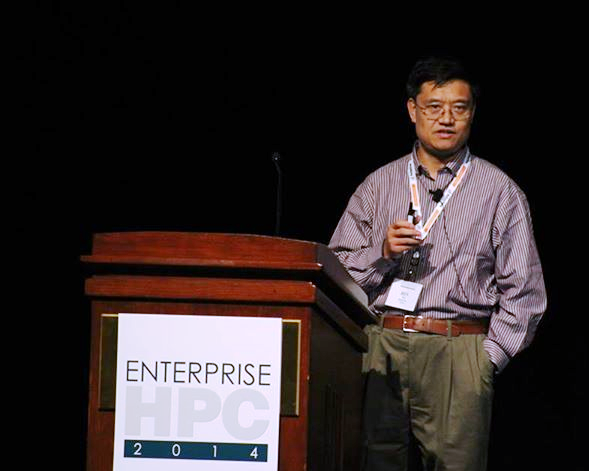
Dr. Ren Wu, a distinguished scientist at Baidu’s Deep Learning Institute (IDL), presenting his keynote at the recent Enterprise HPC event.
The growth is computation power is evident when you consider that a Qualcomm Snapdragon-based Samsung Galaxy Note 3 has the equivalent processing power as the world’s top supercomputer in 1993, the Think Machine CM5/1024. It also stares you in the face when you realize that a pair of GPU-equipped Apple MacPro workstations today has more teraflops than the world’s largest supercomputer in 2000, IBM‘s PowerPC-based ASCI White.
When you take today’s massive computational power, and apply it to the field of deep learning, you suddenly are able to do things that just weren’t possible before. Instead of training a deep neural network on a sample of data, you can basically train it on your entire data set. While Baidu isn’t training its image search function against its entire 2 Exabyte data store, it is employing its massive data set to train machine learning models at scales that were never before imaginable.
According to Wu’s presentation, Baidu is using 10,000 hours of voice data, consisting of more than 10 billion training samples, to train its GPU-powered deep neural network (DNN) HPC cluster. In the image recognition department, its training set is well over 100 million images. Its optical character recognition (OCR) training set also measures in the hundreds of millions, while its click through rate (CTR) model measures in the hundreds of billions. The training sessions run anywhere from weeks to months on Baidu’s clusters, Wu said.
So far, the investment in deep learning has paid off handsomely, delivering a 25 percent reduction in the error rate for speech recognition, a 30 percent error rate reduction for OCR, and 95 percent success rate for facial recognition. Considering that Baidu’s training data sets are growing at a 10x annual rate, the company should be able to continue to fine-tune the delivery of its data services going into the future.
But growing at 10x brings its own set of challenges. At Baidu, the insatiable demand for data storage and processing is putting pressure on the company to expand its data center operations. In 2012, the company responded by committing to spend $1.6 billion on a pair of new data centers in Shanghai. At the time of the announcement, the datacenters were said to be the largest in the world.
The executives at Baidu are on board with making this massive investment in deep learning because they see it as a key competitive differentiator. “When we first deployed our first few deep learning-based applications, we could foresee the need for computation to grow dramatically,” Wu said, adding that the two new data centers will facilitate the delivery of even more deep learning-based applications, including mobile applications that can perform this type of sophisticated image and speech recognition without being connected to Baidu’s servers.
Only a handful of companies could pull off building this melding of hyperscale and mobile, and most of them are tightly clustered in Silicon Valley. Google, for example, has been gobbling up deep learning outfits lately with the goal of bolstering “Google Brain,” which is the unofficial name for Google’s deep learning research project.
But Baidu is showing that big data innovation is occurring outside of the Valley. “What we really want to do here is to push the state of the art,” Wu said. “We want to see 100x or more performance compared to Google Brain, and we count on these things giving us even more competitive advantage.”
Related Items:
Deep Neural Networks Power Big Gains in Speech Recognition
How PayPal Makes Merchants Smarter through Data Mining
Thinking in 10x and Other Google Directives