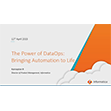
Using Analytic Pipelines to Drain Data Swamps
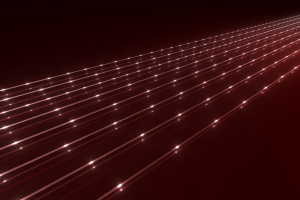
Hadoop excels as a platform for storing vast amounts of data, but it may or may not be the best place for enterprises to run analytic queries. Now enterprises may want to consider an emerging big data architecture that focuses on using analytic pipelines to prevent Hadoop from becoming a big data swamp.
The idea of an analytic pipeline is nothing new. But the concept appears to be gaining steam at troubles with the data lake approach mount.
One firm at the forefront of the big data analytics revolution is Pentaho, a provider of business intelligence and analytics software. Not all of Pentaho’s customers are using Hadoop, but many of them are, and increasingly it’s becoming apparent that they’re not getting the analytic uplift that they had expected, according to Chuck Yarbrough, the big data product marketing manager at Pentaho.
“We’re seeing a lot of people going with these big data architectures, including a data lake,” Yarbrough says. “There’s a good side to data lakes. But if you’re just taking your data and dumping it in a data lake, it can become a data swamp.”
This is particularly true of enterprises that perhaps are taking a very all-encompassing view of what Hadoop will do for their business, he says. While Pentaho supports Hadoop and generates data transformation and ETL processes using Hadoop technologies like MapReduce and Spark, the company realizes that Hadoop is not the only game in town when it comes to enterprise analytics in the real world.
“People who are investing in Hadoop… are finding that when it comes to the end game–which often times an interactive analytics experience for an end-user–having that data sit in Hadoop is not working,” Yarbrough tells Datanami. “It’s the right place to have that data and process that data. But depending on the use cases, it’s not necessarily the right place to put your end user.”
In Pentaho’s view, enterprises that require flexibility and agility in their big data analytics projects are better off investing in a data analytic pipeline strategy. Instead of putting all of one’s eggs into Hadoop, Pentaho sees customers getting a better return by ensuring that the entire analytic process—not just Hadoop but every surrounding source and destination–is sufficiently managed. That means orchestrating the data and making sure that multiple data sources are properly cleansed, transformed, blended, delivered, and governed before the analysis even begins, Yarbrough says.
“The important key there is to deliver [data analytics] with governance so the data is ultimately trusted and provided in a way that people can trust their decisions,” he says. “We tend to view what we do as the managing and automation of a data pipeline as a way to prevent that data swamp from happening.”
Today at the Strata + Hadoop World show in New York City, Pentaho unveiled version 6 of its flagship software suite. The new version furthers the company’s goal of helping customers build analytic pipelines, with a particular focus on helping customers to build and deliver “data services” that leverage existing data storage and analytic resources within their organizations.
Specifically, Pentaho version 6 delivers the capability to blend and virtualize data on the fly; to cleanse virtually any type of data; to push processing down to other parts of the stack; and the capability to track and store data lineage across one or more analytic pipelines. It also sports better integration with IT monitoring tools, including SNMP.
It’s all about exposing a “big blend” of data as a service, Yarbrough says. “The idea is to flexibly enable a blend of data and then exposes that data as a data service,” he says. “The data is coming in from whatever source and all kinds of actions are being taken on that data– integration and transformation and cleansing processes. As part of that path of that pipeline, you’ll have places where data goes to work, like a data lake, but it doesn’t stop there. It may go on to a data warehouse, or something we’re seeing our customers adopt readily, and that is a data refinery.”
This data refinery and data pipeline concept characterizes the work that one particular Pentaho customer is doing. The organization (which the company did not name) is storing every transaction that’s occurring on the stock market, and searching for signs of market manipulation, which is a crime. This data is stored in a Hadoop data lake that’s currently brimming with 7PB of data, Yarbrough says.
But the analysis doesn’t occur in Hadoop. “Doing that is a challenge if it’s sitting in Hadoop. The performance is not necessarily great,” Yarbrough says. “So this is where that idea of the data refinery comes in. They refine it in Hadoop. They bring a smaller data set out, put that data into an analytic engine– in this case Amazon Redshift–and the end-user has immediate access to that data.”
Pentaho 6 will become generally available October 14 during the company’s annual user conference. Pentaho was bought by Hitachi Data Systems earlier this year.
Related Items:
One Deceptively Simple Secret for Data Lake Success
Pentaho Eyes Spark to Overcome MapReduce Limitations
This ‘Bulldog’ CPO Aims to Help Pentaho Grow