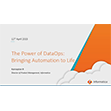
GridGain Adds Persistent Store to In-Memory Platform
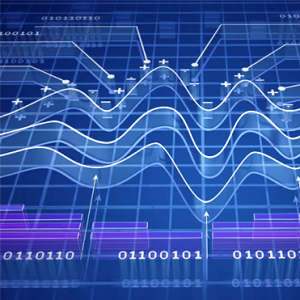
The steady shift to memory-intensive processing continues with the latest release of GridGain System’s in-memory computing platform that seeks to leverage steady advances in memory and storage technologies within a “memory-centric” framework.
GridGain, Foster City, Calif., said this week the 8.1 version of its in-memory system extends SQL capabilities by broadening SQL Data Definition Language features that complement existing Data Manipulation Language and ACID transactional support. DDL is a standard used for commands that define database objects such as tables and indexes.
As for storage, GridGain said its new in-memory platform targets hybrid memory/disk framework that includes a new persistent storage feature. As persistent storage moves to production, especially for emerging tools such as application containers, the high-end version of the latest version also includes a new backup feature called “cluster snapshot” aimed at memory-based architectures running critical production workloads.
GridGain’s latest computing platform based on Apache Ignite is being positioned as an in-memory data grid that can run either with existing relational, NoSQL or Hadoop databases or operate as a standalone transactional SQL database augmented with persistent storage features.
Abe Kleinfeld, president and CEO of GridGain Systems, asserted in a statement that the addition of persistent storage and cluster snapshot capabilities “means it can be used for a broader range of production applications.” The company said it is addressing enterprise requirements that seek to balance operational costs and application performance by adjusting the amount of data kept in-memory.
The GridGain platform is among a raft of storage-based approaches that reflect the shift to high performance computing infrastructure based on more affordable in-memory frameworks. Proponents argue that the use of emerging approaches such as persistent storage helps overcome a major performance bottleneck: disk reads and writes.
GridGain has for example promoted its approach for high-frequency trading applications requiring rapid analysis of huge data volumes. Citing an emerging financial transaction called “spread betting,” Nikita Ianov, GridGain’s founder and CTO claimed in-memory computing provided a roughly 1,000-fold increase in transaction processing speeds.
Indeed, global banks are among GridGain’s largest customers, including Moscow-based Sberbank, which led a $15 million funding round last year. Others banking customers include Barclays and ING.
Along with connecting data stored in relational, NoSQL and Hadoop platforms running cloud-scale applications, GridGain also pitches its in-memory approach as free-standing transactional SQL database the crunches massive data volumes with reduced latencies across scalable clusters of commodity servers.
The persistent storage feature is a distributed ACID and SQL-compliant disk store integrated with Apache Ignite. The company said the approach allows it to integrate its platform as an optional disk layer that can be deployed on spinning disks, solid-state drives, flash memory, 3D Xpoint and other storage-class memory technologies.
Recent items:
Enabling Successful Spread Betting in In-Memory Computing
Russian Bank Funds In-Memory Vendor GridGain