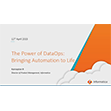
Cloud Tools Rev Up AI Dev Platforms
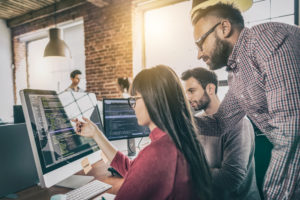
The latest release of analytics startup Dataiku’s platform incorporates application container and orchestration capabilities along with expanded support for deep learning libraries to help launch enterprise AI project at scale.
The data platform vendor said Monday (Sept. 10) the version 5 release of its flagship platform includes Docker container and Kubernetes orchestration tools along with support for visual deep learning libraries and upgrades designed to boost AutoML performance.
Dataiku 5 also includes new documentation features aimed at improving application development among distributed data science teams.
The New York-based data science startup joins a growing list of analytics vendors embracing cloud-native tools like Docker containers and the Kubernetes cluster orchestrator. Dataiku’s spin on containerization includes combining the infrastructure tools with the in-memory processing of Python and R programing languages as well as in-memory model training and scoring.
Dataiku said its in-memory approach allows its updated platform to create Docker images containing code and required libraries that are then automatically deployed to a Kubernetes cluster.
Meanwhile, the visual deep learning capability includes support for the TensorFlow open-source machine learning framework as well as Keras. Keras is a neural network library written in Python billed as a way for users to define their deep learning models directly from a machine learning interface.
The documentation feature targets what Dataiku says is the lack of a digital paper trail for enterprise AI projects that often hinders collaboration and code reuse. The tools are intended to connect developers via a “Discussions” interface that allows data science teams to create, share and edit documentation generated by AI development projects.
Dataiku was again listed by market tracker Gartner as a “visionary” in its recent rankings of data science and machine learning platforms. While slipping slightly over the previous year, Gartner said it remained impressed by the overall ease-of-use of working with the Dataiku DSS platform, and how quickly users can get started with prototyping machine learning projects. It also praised its “pluggable” architecture that lets users incorporate models developed in other environments (like Spark MLLib and the H2O offering). Cautions include the process of moving from development into production, a lack of automation, and pricing.
Founded in 2013, Dataiku announced a $28 million Series B financing round in September 2017.
Recent items:
Winners and Losers from Gartner’s Data Science and ML Platform Report
Why Deep Learning May Not Be So ‘Deep’ After All