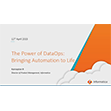
Why You Should Strengthen Your Retail Analytics Strategy with Multiple Versions of the Truth
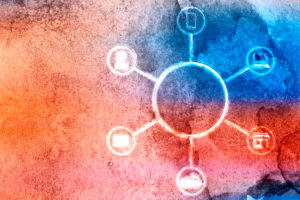
(Semisatch/Shutterstock)
With an influx of data into retailers’ environments—from e-commerce, social media, in-store data and supply chain technology, to name a few new sources—structuring how to interpret that data and then act on it to create unique and personalized customer experiences requires a different approach to business intelligence (BI). While a single version of truth (SVOT) has long been the holy grail of analytics professionals, the likelihood of retailers understanding the nuances of their customers’ habits and desires through a single lens is not only impractical — it’s also problematic.
For retailers, being able to connect with customers and personalize the shopping experience is a key differentiator in a highly competitive industry. To predict customer behavior and nudge them toward recommended products requires a sophisticated analytics approach. You can’t be one of the 87 percent of organizations with low levels of analytics maturity and meet the expectations of today’s retail consumers.
Even those retailers moving up the analytics maturity model are struggling with a proliferation of data sources, data platforms, BI tools and an organizational culture that isn’t aligned to maximize the value of their analytics ecosystem. The new analytics paradigm for retailers increasingly resembles what business intelligence leaders have been railing against for years: multiple data silos, no single system of record and uncertified data. Frankly, it looks like the Wild West. The knee-jerk reaction to this phenomenon is to control it, ratchet it down and force all data flows into a single, governed process. It’s a natural response—we’ve all been in important meetings where business discussions have devolved into fighting about the numbers.
Although well-intentioned, a strategy designed to control data has the opposite effect in most organizations. There’s simply too much content and too much business demand to execute a single-pipeline, single team, centralized data analytics strategy. Organizations that focus on an SVOT strategy, ironically, end up with a reality they were trying to avoid in the first place: data silos, shadow IT projects initiated without the knowledge of IT leaders, proliferation of content, and organizational risk.
Why? Because just as water traveling through solid rock toward the ocean always finds a way to get there, analysts who needed answers days ago will always find a way to get them—even if that means buying an Alteryx license with a corporate credit card.
Retail Customers Live in a Multiverse
It’s no longer shocking to see stats on how ubiquitous data has become over the past decade–more than 2.5 quintillion bytes of data are now generated each day. That means that even the most optimized technical teams will struggle with SVOT.
This challenge will only be amplified as the amount of data available to retailers increases: The market for retail Internet of Things (IoT) sensors, RFID tags, beacons and wearables is projected to grow 23% annually through 2025, which will generate data needed for targeted customer experiences and optimized operations. As retail consumers increasingly live and shop across multiple channels, a new strategy for analytics is needed to take advantage of all that additional data.
Single data pipelines that slow learning abstraction and decision-making based on those insights are not the right fit for this new paradigm. A single data pipeline prevents analytics from delivering insights at the pace needed by line-of-business decision makers.
In an SVOT world, employees often lose patience with the process and attempt do-it-yourself strategies with data. An environment where marketing, sales, demand planning, supply chain, operations and finance each apply their own tools, filters and data-modeling decisions will result in a multitude of interpretations, even if they start from the same pile of data.
The Case for Multiple Versions of the Truth
Technical leaders should embrace the chaos and lean into multiple versions of truth (MVOT)–after all, MVOT is going to occur despite the most valiant control efforts. This simple act of acceptance can be liberating and productive for organizations. It can also create a much-needed culture of collaboration, which is the true answer to this challenge.
Once an understanding and acceptance of MVOT is achieved, leaders can then focus on data democratization strategies to get the most out of their analytics programs. The goal should be to shift from SVOT to MVOT based on a culture of collaboration that is self-regulating. Ultimately, the subject matter expertise that’s required to think critically about data sits within the lines of business, and this strategy allows retailers to leverage that knowledge to increase the overall ROI of their analytics programs.
Six Steps to Achieve Data Democracy
To enable an analytics program based on data democracy that allows for MVOT, retailers must create an ecosystem that drives collaboration and ensures precision. Finding the right balance of governance to ensure agility while preventing your analytics function from becoming the Wild West is the trick. Here are a few ideas on how to achieve it:
-
Implement a self-service analytics strategy. Traditional analytics programs place the bulk of the management and maintenance workload onto IT teams. This often leaves business units dependent on IT anytime they need data processed or manipulated, which slows down time-to-insight. To get line-of-business staff to take ownership of analytics, you must first enable them to operate as self-sufficiently as possible. Your objective should be to develop an analytics program that is as streamlined, easy-to-use and accessible for non-data scientists as possible. This usually includes adopting self-service platforms and establishing data sandboxes for analysts to explore new data safely. It also requires an investment in training programs.
- Enable a data governance model to support self-service analytics. With new users comes the risk that data will be processed or manipulated incorrectly. Head off any potential issues down the road by setting a deliberate governance model focused on documenting data sources and the choices made with each data set. It should enable data sharing but thoughtfully consider how, when and with whom it should be shared. And it should lay out your company’s data ingestion, certification and modeling protocols to ensure that all users can produce accurate and timely insights. There should be a development lifecycle established, even if lightweight, to ensure that content is peer reviewed and cataloged. This depoliticizes analytics results and guides internal discussion around them when, for example, sales reports certain numbers that contradict those reported by demand planning.
- Stop the platform turf wars. Many organizations get hung up on turf wars over their favorite analytics tools. Most eventually decide to standardize and force adoption on a single set of tools, but attempts to force a single perspective will typically backfire and drive a dysfunctional culture, eroding the trust that the ecosystem needs to be successful. This will undermine your business’ ability to find key insights that could otherwise drive ROI. Instead, work with each business unit to identify the best way to share data that makes the most sense for its analytics needs and staff experience level. Create internal user groups so people can learn about the various tools and methodologies the organization is using. You might be surprised how quickly alignment on tools and approaches happens organically.
- Create a center of excellence. Creating a self-sustaining analytics culture is a significant investment, and to be successful, you need a center of excellence (COE) to help facilitate the process. These are not command-and-control organizations. COEs are either virtual or dedicated teams that are service providers to any and all members of the internal analytics community. They educate, help align best practices and facilitate processes such as platform evaluations and lifecycle management.
- Set up a data catalog. Create a catalog that democratizes data by enabling users to see and gain access to data as it is added. The data catalog should have a portal with a social media-like experience that enables users to mark favorite data sets, certify data, share them with other users and easily interact with data. This will vastly increase collaboration across teams and ensure that all team members have access to the information they need, when they need it. The best data cataloging platforms integrate with multiple databases and BI tools.
- Start with the end (production) in mind. There’s an old joke in analytics: If you ask a room of data scientists to raise their hands if they’re working on an exciting use case, all hands will soon be in the air. If you ask again how many of the same data scientists have their use case deployed into production, no one will raise their hands. The lesson of this tale is that it’s not enough to simply tinker with data. You must begin with the end in mind, which involves thinking though how each analysis will operate in production to serve the original purpose. There is very little ROI achieved with one-time insights communicated in a PowerPoint, regardless of how brilliant.
The lack of agility and long-term scalability associated with unlocking SVOT make it an approach that’s no longer viable in retail, or any customer-centric industry for that matter. By instead implementing a collaborative analytics program focused on MVOT, retailers can make better use of their internal expertise, reduce time-to-insight and better adapt to new technologies.
About the author: Brad Jackson is vice president of digital intelligence at ProKarma, where he helps the world’s most recognized brands escalate growth. He has nearly two decades of experience delivering analytics solutions for leading organizations.
Related Items:
How Walmart Uses Nvidia GPUs for Better Demand Forecasting
How Brick and Mortar Retailers Can Avoid Getting Amazoned With Big Data
Unlocking Retail’s Big Data Opportunity