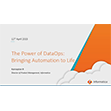
Giving DevOps Teeth To Crunch Down on AI Ethics Governance
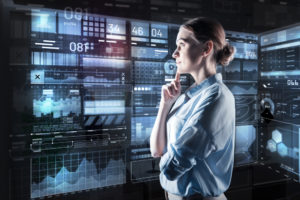
(YAKOBCHUK-VIACHESLAV/Shutterstock)
AI ethics is definitely trending. I’ve seen the phrase in my reading and heard it trip from the tongues of professional acquaintances many times in the past several months.
Management fads come and go, and I wonder whether AI ethics might be one of them. In much the same way that product quality deficiencies triggered the ISO9000 fever of the 1990s and corporate malfeasance stoked the Sarbanes-Oxley mania of the 2000s, anxieties surrounding AI’s misuse are now the focus of much soul-searching in business and technical circles.
Fads fade when society realizes they may have been overblown or that they proposed changes in the status quo that didn’t make sense beyond a niche subculture. As we examine the anxieties behind the ethical AI movement, we must ask whether they’ve been hyped out of proportion by mainstream culture. We must also ask whether the approaches being proposed for instilling ethics in the business AI development and operations are truly taking hold or are likely to ensure that ethically dubious AI applications never see the light of day.
One of the things that concerns me about today’s AI ethics mania is the top-down nature of how it’s being addressed by corporate management. What I’d like to see is working data scientists building ethics-assurance safeguards into their development and operations workflows. Instead, it appears that upper-echelon business executives are driving the show through committees, working groups, and other talk-intensive business-suit exercises of a non-binding, advisory nature.
Reminiscent of how the business world half-heartedly implemented Total Quality Management principles a generation ago, this recent Brookings Institution report calls for the following top-down governance tactics:
- Hire a company AI ethics officer.
- Establish a company-wide AI ethics oversight board that also includes customers and other stakeholders.
- Publish an AI code of ethics.
- Conduct regular AI ethics audits.
- Survey employees, customers, and stakeholders on AI ethics matters.
- Require employees to receive AI ethics training and certification.
- Institute AI ethics whistle blowing and appeal processes.
- Organize an AI ethics center of excellence.
That’s all well and good. But it all strikes me as a well-meaning attempt to add new layers of red tape, meetings, and documentation that will have little input into AI development and operations processes. To the extent that these procedures become binding mandates on the AI development process, the bureaucratic overkill could foster cynicism around the need for AI ethics safeguards of any sort. Through the “cry wolf” effect, bureaucratic overreach could also weaken the business case for addressing the privacy, bias, and other ethically suspect impacts that AI’s misuse can indeed foster.
In a similar vein, AI ethics initiatives tend to dilute their practical impact through excessively broad scopes. As evidenced by popular articles such as this, AI ethics discussions often overshoot their mark by obsessing over a Pandora’s box of evils which this technology is ostensibly exacerbating. If you’re an enterprise trying to prioritize your investments in AI ethics safeguards, it’s best not to concern yourself with extraneous topics such as the technology’s potential uses in killing jobs, developing killer robots, and perfecting mass surveillance. Instead, you should focus on those AI-driven outcomes that have some bearing on core business objectives and customer engagement concerns, such as protecting privacy and eliminating racial biases in sales and marketing.
If you’re truly concerned with mitigating the ethics-related issues surrounding AI, try not to spend too much time obsessing over geopolitical and humanitarian concerns that are, as they say, “above the pay grade” of the working data scientist. If you truly want to ensure that ethics-friendly AI apps become standard in your company, the appropriate governance controls must be baked into the tools and platforms that drive development and operations workflows.
As you incorporate ethics safeguards into the AI pipeline, please consider the following guidance:
- Incorporate a full range of regulatory-compliant controls on access, use, and modeling of personally identifiable information in AI applications.
- Ensure that developers consider the downstream risks of relying on specific AI algorithms or models—such as facial recognition—whose intended benign use (such as authenticating user logins) could also be vulnerable to abuse in “dual-use” scenarios (such as targeting specific demographics to their disadvantage).
- Instrument your AI DevOps processes with an immutable audit log to ensure visibility into every data element, model variable, development task, and operational process that was used to build, train, deploy, and administer ethically aligned apps.
- Institute procedures to ensure the explainability in plain language of every AI DevOps task, intermediate work product, and deliverable apps in terms of its relevance to the relevant ethical constraints or objectives.
- Implement quality-control checkpoints in the AI DevOps process in which further reviews and vetting are done to verify that there remain no hidden vulnerabilities—such as biased second-order feature correlations—that might undermine the ethical objectives being sought.
- Integrate ethics-relevant feedback from subject matter experts and stakeholders into the collaboration, testing, and evaluation processes surrounding iterative development of AI applications.
Ideally, these ethics safeguards should be automated, enforced, and monitored at every stage in the AI DevOps lifecycle. Bear in mind that, though there are a growing range of AI development, workflow, and collaboration tools on the market, few if any have been explicitly designed with cradle-to-grave ethics guardrails. However, that shouldn’t stop you from pressing vendors to add these capabilities to their offerings.
Furthermore, you may have acquired key AI applications from software as a service providers and outsources of all types. If so, you need to ask them to provide full disclosure of their own practices—such as ethics officers, oversight boards, codes of conduct, audit logs, and automated controls—for ensuring their alignment with your organization’s AI ethics principles.
What you’re likely to find is that your AI applications and tool vendors are still trying to bring their ethics-assurance frameworks into coherent shape. Everybody—even the supposed experts—are groping for a consensus approach and practical tools to make ethics assurance a core component of AI DevOps governance.
About the author: James Kobielus is SiliconANGLE Wikibon‘s lead analyst for Data Science, Deep Learning, and Application Development.
Related Items:
Let’s Accept That AI Leadership Is Everywhere
Predictions for Application Development in 2019