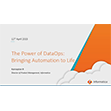
Using AI Sound Analysis to Protect the Rainforest
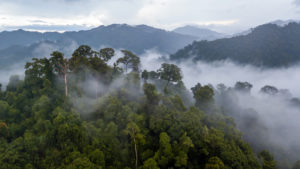
With climate change accelerating and the Amazon burning hotter than it has in years due to rampant deforestation, conservationists are racing to find creative ways to apply technology to protect the Earth’s rainforests. Typically, much of this work has focused on inferences from high-resolution satellite imagery. But recent research from Huawei Cloud, Futurewei and Rainforest Connection focuses on leveraging another dataset: sound.
“Compared with image data which is limited by field of view and area covered with dense understory such as the rainforest,” the researchers wrote, “audio data can be a good fit in the sense of ease and robustness of data acquisition, low data volume and high information density.” After all, the authors note, some sound classification models have already outperformed humans when analyzing urban soundscapes – so why not apply the same technology to rainforests?
In their paper, the authors propose new convolutional neural network (CNN) models for classifying environmental sounds. Existing CNN models, they explain, are situated in domains that are too distinct from environmental sound classification, and might not offer the desired computational efficiency.
First, the authors propose a modified version of the “VGGish” model broadly used in audio recognition. Rather than its stock 72.1M parameters, the “Aug-VGGish” model utilizes only 4.7M parameters and includes batch normalization after each convolutional layer, among other changes. Second, they propose the use of a fully convolutional network (FCN) approach (traditionally used for vision tasks) for audio recognition, calling the resulting 18.7M-parameter model “FCN-VGGish.” These models, the authors say, are better able to balance capacity and capability, as well as improving model performance using transfer learning techniques.
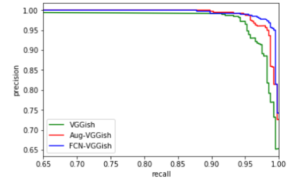
Accuracy of chainsaw sound classification compared among the three models. Image courtesy of the researchers.
With the models built and tested, the researchers applied them to 22,000 real-world rainforest audio recordings collected on-site using smartphones. The one-second clips were annotated by Rainforest Connection to denote whether or not a chainsaw could be heard in the clip. They found that both Aug-VGGish and FCN-VGGish outperformed the stock VGGish model, with FCN-VGGish achieving over 95% recall even at high precision constraints.
The authors hope that these models, which they applied to smartphone recordings, might be more realistically scalable than other approaches. “Deploying AI-powered systems in rural areas faces a wide variety of critical restrictions, such as very limited power supply, poor connectivity, and harsh conditions,” they wrote. “We need to utilize a practical yet effective modality to sense the environment and provide informative data to support decision making.”
The team hopes to expand the research in the future, including implementation of advanced learning techniques to improve audio recognition and examination of other rainforest sounds, such as spider monkey habitat modeling. “With our NGO partner,” they wrote, “we are making cloud-based AI solutions for rainforest conservation a reality.”