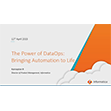
2019: A Big Data Year in Review – Part One
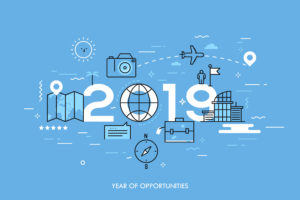
(Andrew-Krasovitckii/Shutterstock)
At the beginning of the year, we set out 10 big data trends to watch in 2019. We correctly called some of what unfolded, including a renewed focus on data management and continued rise of Kubernetes (that wasn’t hard to see). But a lot of stuff transpired in our little neck of the big data woods that was completely unpredictable. As they say in football, that’s why we play the game.
Here are some of the most important stories we’ve covered in Datanami for the year, in reverse chronological order.
2019 started out promising enough for Cloudera, which completed its $5.2-billion acquisition of rival Hortonworks on January 3. While details in creating a combined Hadoop distribution were to be worked out, the new Cloudera was keen to position itself for emerging “Edge to AI” workloads. But storm clouds around Hadoop were building, as we would see later in the year.
The United States has suffered from a data science skills shortage for a number of years, and that continued in 2019. Nearly half of companies in one survey say they were looking to hire data scientist or data engineers. The survey also noted large geographic differences in data science salaries, with the San Francisco leading the way.
AWS dominates the public cloud company like no other company, and for good reason: No other cloud provides the depth and breadth of storage and computational services that AWS does. But will customers become trapped if they build on AWS services? That’s a real concern, experts say. The solution: Build on AWS with your eyes open.
What you can’t see can hurt you, as any cybersecurity expert will tell you. That’s why we’re seeing an increase in use of unsupervised machine learning techniques to create a fuller picture of security risks in real world, particularly in financial services, where unsupervised ML can find patterns hidden in very large data sets.
There’s a natural ebb and flow to data. Sometimes it’s more centralized in an organization, and sometimes it’s more dispersed. The folks at Gartner saw data’s natural gravity taking over with “analytics hubs,” where large amounts of data are consolidated for traditional analytics, machine learning, and graph analytics use cases.
We’re several years into deep learning’s “Cambrian Explosion,” which gave us a fantasic array of new technologies. But now there are signs that the various deep learning lifeforms are coalescing into a common stack, with the TensorFlow and PyTorch frameworks, Kubernetes orchestration layer, Juptyer visual interface, and Kubeflow or Airflow coordinators. (It all runs on Linux, of course.)
Every year, Datanami recognizes a dozen of the most influential voices in big data. In February, we unveiled our 2019 People to Watch, which could be the best group yet.
Data is the key ingredient to every machine learning project. So why do so many organizations overlook the importance of cleaning and labeling training data? Nobody knows for sure, but one thing is certain: the situation is developing a market for third party data labeling services.
2019 marked a key year in big data architectures, as organizations moved data into cloud repositories at unprecedented rates. The soaring popularity of S3 and other S3-based object stores continued to chip away at on-premise HDFS clusters, which began to look a bit long in the tooth this year.
AI was the name of the game in 2019, as companies looked to leverage their data for the maximum gain. According to one study, AI helped strong companies extend their leads over less-capable competitors.
Think you have demanding operational data requirements? Consider The Trade Desk, which computes 9 million advertising impressions per second with a latency of 40 microseconds. The company, which spends $90 million per year on hardware, uses an array of big data technologies to achieve that.
Apache Hadoop celebrated its 10th birthday in 2016, and in 2019 it was Apache Spark’s turn to turn 10. We looked back on the improbable rise of the technology that was explicitly designed to replace MapReduce, but has become such an indispensable tool for data scientist and engineers alike.
If you’re looking for a pragmatist in big data, Doug Cutting is your guy. The co-creator of Hadoop and Cloudera chief architect never expected that technology to take off like it did. And in a year that saw Hadoop struggle, Cutting’s assessment, delivered to Datanami at the Strata Data Conference in San Francisco, that nothing is poised to replace Hadoop for large-scale, on-premise processing should be considered.
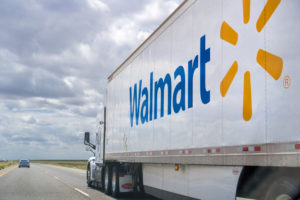
Wal-Mart optimized its weekly sales forecast using an AI setup running on GPUs (Sundry Photography/Shutterstock)
Nobody has dominated the business analytics segment like SAS. And with its commitment to invest $1 billion in AI over the next three years, the odds of SAS continuing its preeminent position increased.
The mother of all AI logistics use cases could be at Wal-Mart, which uses an array of machine learning algorithms running on dozens of Nvidia GPUs to create weekly forecast sales of over 100,000 products at over 4,700 stores. When it’s all said and done, the company reported that the machine learning setup boosted forecast accuracy on the order of 1.7%. Not bad for the world’s largest company.
Is AI becoming the fourth pillar of the scientific method, following the experimental method, theoretical reasoning, and computer simulation? That’s what Nvidia CEO Jensen Huang claimed at his company’s GTC event in March. (An impromptu Twitter poll subsequently failed to validate the claim.)
Why is the cloud so popular? Some say because it’s easy to get started. Others say it’s adaptable to one’s needs. When it comes to cost, however, Lyft’s $8-million-per-month AWS bill would suggest that saving money is not one of cloud’s greatest attributes.
Big data. High performance computing. AI software. These are the three critical elements that organizations are using to differentiate themselves from competitors, a digital holy trinity, if you will. They also happen to be the core foci of Tabor Communications Inc.’ publications, Datanami, HPCwire, and EnterprsiseAI, which joined forces for TCI’s Advanced Scale Forum event in Florida this April.
What’s the best stream processing system and messages busses for your particular use case? We researched and dug into the most popular stream processing frameworks, from Apache Storm Apache Flink, as well as the top real time message busses to help you understand how they’re intended to be used.
Related Items:
10 Big Data Trends to Watch in 2019
Industry Speaks: Big Data Prognostications for 2019
AI Prognostications Plentiful for New Year