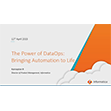
Automatic Data Labeling Gains Momentum with New IBM and Labelbox Announcements
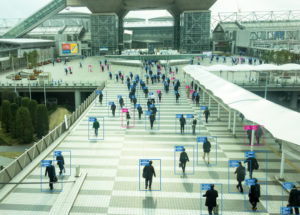
Data is powerful, but labeling data makes it useful. Labeled data (data that has been appended with informative tags about its contents – say, whether a photo is of a person or an animal) can be used to quickly train machine learning models for identification. Furthermore, automated, AI-driven labeling tools can help to speed the initial process of labeling the data. Now, a pair of back-to-back announcements from IBM and Labelbox are signaling new momentum in the data labeling tool space.
IBM made the first of the two announcements: a new automated labeling tool called “Cloud Annotations.” The tool, which is open-source and accessible on GitHub, allows users to feed 200-500 hand-labeled images into it, after which AI takes the wheel and automatically labels the remaining image set. Cloud Annotations also allows for real-time collaboration as well as cloud data storage and access through IBM’s public cloud.
“Cloud Annotations is a fast, easy, and collaborative open source image annotation tool,” wrote Nicholas Bourdakos, developer advocate for IBM Cognitive Applications. “The Cloud Annotation auto labeling feature is currently live on GitHub, available for anyone to use and take advantage of as a massive time saver. Start using the tool and let us know what you think!”
Then, not even a week later, another announcement: Labelbox, an AI-powered startup founded in 2018, closed its $25 million Series B funding round, bringing it to $39 million in venture funding from the likes of First Round Capital, Gradient Ventures and Kleiner Perkins. Labelbox bills itself as defining a new category of software: “training data platform.” The company offers tools for label editing, bath and real-time labeling, collaboration, quality review and analytics, as well as an optional dedicated labeling workforce. Labelbox touts its use by more than a hundred companies, which they say span industries ranging from agriculture (for weed identification) to sports analysis (for tracking players).
“If GitHub has become the platform for managing and developing software code, then Labelbox has the potential to fill a similar role for data in the AI/ML world,” said Peter Levine, general partner at Andreessen Horowitz and leader of the Series B funding round. “We see Labelbox becoming the single source of truth for defining, storing, and accessing training data across an entire organization. We are thrilled to partner with company founders Manu, Brian, Dan, and the team to help them realize their vision.”
Labelbox found its origin story when Manu Sharma, its cofounder, was working at Planet Labs, which found itself needing to create and manage training data in the absence of an off-the-shelf solution. “A lot of this tooling was being built from scratch,” said Sharma. “It seemed crazy to us that data scientists were building core infrastructure in order to get started with AI.” Now, it seems, the race is on.