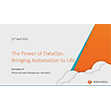
Revolutionizing Data Collaboration with Federated Machine Learning
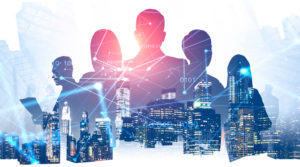
(ImageFlow/Shutterstock)
We now live in a world that’s becoming more data-driven every day. Organizations across a wide range of industries are using artificial intelligence (AI) and machine learning (ML) technologies to tap into complex data sets, unearth valuable insights and drive innovation. From healthcare and government to the financial sector and beyond, advanced data science models and big data projects are unlocking insights that can deliver everything from novel approaches to preventing and treating disease to highly effective financial fraud detection and more.
But these projects aren’t without their challenges. Organizations looking to embark on data collaboration initiatives must overcome obstacles such as data ownership issues, compliance requirements for a variety of regulations and more. In today’s data-filled world, ensuring privacy and security is paramount, and the measures to which organizations must go to achieve this can make collaborative data science difficult. The potential consequences of sustaining any kind of privacy or security breach (noncompliance, fines, reputational damage, etc.) can cause organizations to shy away from sharing data sets that could spark the next life-saving medical treatment or momentous public service program.
Solving Big Data Collaboration Problems
Luckily, organizations across many industries are recognizing just how much upside we’re leaving on the table if valuable data sets remain siloed. As such, they’re advocating for new approaches to running algorithms on data from various parties that can prevent the sources from being compromised by or shared with outside entities.
An early approach that attempted to solve these issues came in the form of a centralized data aggregation model. This involves migrating each collaborator’s data sets to a single aggregation engine in a private, inaccessible execution environment within a processor. The intent here was to ensure that each party’s data sets remained private and that only the results of the query could be shared. Unfortunately, this approach comes with numerous challenges – from unmanageable data set sizes to incongruent file formats among participating parties that can make aggregation untenable and more.
Needless to say, it quickly became evident that this early attempt at solving the world’s major data collaboration problems had to be improved upon. That’s where “Federated Machine Learning” comes in.
What is Federated Machine Learning?
A distributed machine learning method first introduced by Google about five years ago, Federated Machine Learning offers tremendous advantages when it comes to privately and securely enabling model training against large pools of data from multiple entities. It takes the opposite approach of the previous technique, meaning Federated Machine Learning will bring aggregation to the data sources, rather than requiring all participating organizations to move their data sets to a centralized compute environment for aggregation. Processing takes place onsite at each individual organization’s location and only the query results are delivered back to the core compute environment where the collective model is then updated.
This decentralized method alleviates many common privacy concerns associated with data collaboration. But, what about security against compromised or stolen data due to weaknesses in the aggregation model, vulnerabilities in communication links, etc.?
The Role of Hardware in Federated Machine Learning
Organizations participating in big data collaboration projects require security layers across the entire compute experience down to the hardware level. When deployed at both individual participants’ edge nodes, as well as within the core aggregation engine, hardware-based Trusted Execution Environments (TEEs) can help secure model training and aggregation while shielding code and data from leakage or compromise from end-to-end across the entire system. This means the various parties involved in the project can rest assured that both their respective data sets and the machine learning model are kept private and secure.
Realizing the Benefits of Decentralized Data Collaboration
Federated Machine Learning offers a viable method for ensuring data privacy and security throughout data science initiatives, but we’re still a ways out from its ubiquitous commercial adoption. For this to happen, public and private enterprises, governments, technology trailblazers and others within the global community will need to cooperate alongside one another to develop inclusive standards and common practices for decentralized data sharing. If we can make this vision a reality, over the next decade, Federated Machine Learning enabled with TEEs will likely bring about a Cambrian explosion of innovative breakthroughs across every data science discipline and industry.
About the author: Nikhil M. Deshpande is the Director of AI and Security Solutions Engineering in the Data Platforms Group at Intel. In prior roles, he led silicon security strategic planning in the Data Center Group as well as managed numerous security technologies research in Intel Labs including privacy preserving multi-party analytics. Nikhil has spoken at numerous conferences and holds 20+ patents. He holds M.S. and Ph.D. in Electrical & Computer Engineering from Portland State University. He also has M.S. in Technology Management from Oregon Health & Science University.
Related Items
How Badly Will COVID-19 Hurt the Data Privacy Movement?
Yes, You Can Do AI Without Sacrificing Privacy
Keeping Your Models on the Straight and Narrow