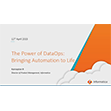
AWS Leans on Custom Silicon for Processing Advantage
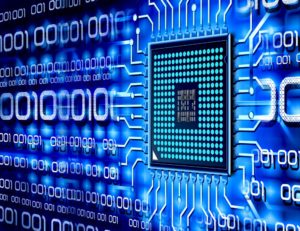
(Robert Lucian Crusitu/Shutterstock)
You may know Amazon Web Services as a leading provider of public cloud services. But it’s also investing substantial sums into designing its own line of custom chips that can save customers a lot of money compared to Intel X86 and Nvidia GPU processors, for general purpose, as well as analytical and AI workloads
According to Raj Pai, vice president of AWS EC2 product management, tells Datanami that AWS customers will see a 40% price-performance increase when they adopt the sixth-generation EC2 instance, which is powered by a custom-designed Graviton2 processor that’s equipped with 64-bit Arm Neoverse cores.
“A number of our customers are actively migrating their workloads from X86 to Graviton,” he says, including SmugMug, Intuit, and Snap. “It’s rare you see this sort of advantage in the industry where a simple migration can save you 40% on TCO.”
The migration isn’t plug-and-play for all applications, Pai says. But it should be a fairly simple move for applications designed atop a “modern framework,” such as PHP, he says.
“Most frameworks [now] are built with interpreted languages, so these packages just kind of run, and you don’t even have to do a recompile,” he says. “Our largest customers, a good portion of their workloads are with these modern frameworks, and they’ve been able to start running on Graviton in a matter of hours or days.”
Other applications, notably databases like Microsoft SQL Server and Oracle’s eponymous database, may take a bit longer to get running on Graviton, he says. “If you’re using more legacy applications that were built in C or C++, typically with those you’re going to have to do a recompile.”
The company’s popular Amazon Redshift and Amazon Athena data warehousing services, which are based on the ParAccel MPP database and Presto SQL query engine, respectively, don’t run on Graviton yet. But they will, and when they do, customers will enjoy comparable price-performance improvements.
“As these services move to Graviton–and we’re still in the process of migrating a lot of the services over–you’re going to see improvements in those dimensions, as far as performance and cost, mapping to the improvement we have in the silicon,” Pai says.
So how did AWS make the shift from X86 to Arm-based processors so easily? According to Pai, it’s all a result of the company’s adoption of the Nitro system, in which various server components, like the virtual machine, storage, and the processors themselves, are re-packaged as cards that be plugged in anywhere and that effectively shield them from the overall complexity of running in an environment as advanced as EC2.
“Because we are able to encapsulate a lot of what it takes to operate a virtual machine and take it off the hardware, it does make it a lot simpler to introduce new architecture technologies,” he says.
Nitro was critical in accepting Apple Mac instances into EC2, as the company announced during the recent re:Invent conference. It’s also what enabled the company to bring AMD-based GPUs into the EC2 mix to complement Nvidia GPUs, as well as its custom AWS Inferentia ASIC, which was launched a year ago and which boasts a price-performance improvement of on machine learning inference by 35% to 40% versus a typical GPU.
And Nitro will also be core to AWS’s next custom chip, called AWS Trainium. The chip, like AWS Inferntia and Graviton2, was developed by Annapurna Labs, the Israeli chipmaker that AWS acquired in 2015 for around $350 million. (Nitro is also an Annupurna Labs creation.)
Pai says AWS “will be delivering a chip focused on training which will offer the best price performance for training workloads for deep learning models, including those in MXNet and Tensor[Flow], towards the end of next year.”
Considering AWS’s position at the head of the public cloud table, it really shouldn’t come as a surprise that the company is embarking into the previously cloistered world of microchip design.
“When you think about it, we have way more knowledge of how cutometers run their workloads in the cloud than anyone else,” Pai says. “So we’re in a pretty unique position to optimize our silicon to meet those needs.”’
The ability to see exactly how AWS’s customers are running their applications enables AWS to eliminate a lot of unneeded components that otherwise might go into a general-purpose chip, Pai says.
“When we built Graviton, we knew that we could hyper-focus on what are those workloads that people are bringing to the cloud,” the AWS VP says. “You don’t have to go to the lowest common denominator, like a lot of chip manufacturers do today. If you’re building general purpose chip, you have to build it to work on a phone and a laptop and a server and a workstation…You keeping adding IPs to that chip so at the end of the day, there’s a lot of transitions in there that may not be used for the vast majority of workloads. And you’re paying for that. It’s part of the cost of the chip.”
That doesn’t mean the company is abandoning traditional processor partners. On December 1, AWS CEO Andy Jassy announced that, in mid 2021, AWS will make generally available new EC2 instances that use the Intel Habana Gaudi AI accelerators. The company said that an 8-card Gaudi solution can process about 12,000 images-per-second training the ResNet-50 model in TensorFlow, which will deliver a 40% price-performance advantage over standard GPUs. And you can also get access to the latest, greatest GPUs from Nvidia, the A100, powered by Ampere.
But as deep learning becomes more important for AWS customers, don’t be surprised to find AWS doing its best to capture more of that market on processors of its own design.
“I think it’s imperative that we invest in silicon and our infrastructure because the opportunity ahead of us is much bigger than the one behind us,” Pai says. “Five to six years ago, when I asked about deep learning for our top customers, a few would raise their hand. But now it’s become a business imperative. Everyone is doing more deep learning training. They have to, to stay competitive. So getting the price-performance down on those workloads is one of our biggest business imperatives on the AWS side.”
Related Items:
AWS Launches Managed Services for Grafana, Prometheus
AWS Bolsters SageMaker with Data Prep, a Feature Store, and Pipelines
Editor’s note: This story was corrected. Neither of AWS’s new chips, Inferentia nor Traninum, are based on Arm. Datanami regrets the error.