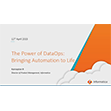
To Bridge the AI Ethics Gap, We Must First Acknowledge It’s There
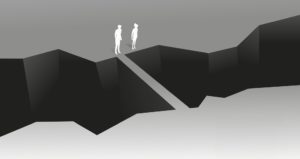
(bookzv/Shutterstock)
Companies are adopting AI solutions at unprecedented rates, but ethical worries continue to dog the roll outs. While there are no established standards for AI ethics, a common set of guidelines is beginning to emerge to help bridge the gap between ethical principles and the AI implementations. Unfortunately, a general hesitancy to even discuss the problem could slow efforts to find a solution.
As the AI Ethics Chief for Boston Consulting Group, Steve Mills talks with a lot of companies about their ethical concerns and their ethics programs. While they’re not slowing down their AI rollouts because of ethics concerns at this time, Mills says, they are grappling with the issue and are searching for the best way to develop AI systems without violating ethical principles.
“What we continue seeing here is this gap, what we started calling this the responsible AI gap, that gap from principle to action,” Mills says. “They want to do the right thing, but no one really knows how. There is no clear roadmap or framework of this is how you build an AI ethics program, or a responsible AI program. Folks just don’t know.”
As a management consulting firm, Boston Consulting Group is well positioned to help companies with this problem. Mills and his BCG colleagues have helped companies develop AI programs. Out of that experience, they recently came up with a general AI ethics program that others can use as a framework to get started.
It has six parts, including:
- Empower Responsible AI Leadership – Appoint a leader who will take responsibility and give her a team;
- Develop principles, policies, and training – These are the core principles that will guide AI development;
- Establish human and AI governance – The system for reviewing adherence to principles and for participants to voice concerns;
- Conduct Responsible AI reviews – Build or buy a tool to conduct reviews of AI systems at scale;
- Integrate tools and methods – Directly imbuing ethical AI considerations into the AI tools and tech;
- Build a test-a-response plan – The system for responding to lapses in principles and for testing. You can read more about BCG’s six-part plan here.
Steve Mills, AI Ethics Chief for Boston Consulting Group,
The most important thing a company can do to get started is to appoint somebody to be responsible for the AI ethics program, Mills says. That person can come from inside the company or outside of it, he says. Regardless, he or she will need to be able to drive the vision and strategy of ethics, but also understand the technology. Finding such a person will not be easy (indeed, just finding AI ethicists let alone executives who can take this role is no easy task).
“Ultimately, you’re going to need a team. You’re not going to be successful with just one person,” Mills says. “You need a wide diversity of skill sets. You need bundled into that group the strategists, the technologists, the ethicists, marketing–all of it bundled together. Ultimately, this is really about driving a culture change.”
There are a handful of companies that have taken a leadership role in paving the way forward in AI ethics. According to Mills, the software companies Microsoft, Salesforce, and Autodesk, as well as Spanish telecom Telefónica, have developed solid programs to define what AI ethics means to them and developed systems to enforce it within their companies.
“And BCG of course,” he says, “but I’m biased.”
Rooting Out Bias at Salesforce
As the Principal Architect of the Ethical AI Practice at Salesforce, Kathy Baxter is one of the foremost authorities on AI ethics. Her decisions impact how Salesforce customers approach the AI ethical quandary, which in turn can impact millions of end users around the world.
So you might expect Baxter to say that Salesforce’s algorithms are bias-free, that they always make fair decisions, and never take into account factors based on controversial data.
You would be mistaken.
“You can never say that a model is 100% bias free. It’s just statistically not possible,” Baxter says. “If it does say that there is zero bias, you’re probably overfitting your model. Instead, what we can say is that this is the type of bias that I looked for.”
To prevent bias, model developers must be conscious of the specific types of bias they’re trying to prevent, Baxter says. That means, if you’re looking to avoid identity bias in a sentiment analysis model, for example, then you should be on the lookout for how different terms, such as Muslim, feminist, or Christian, affect the results.
Other biases to be on the lookout for are gender bias, racial bias, and accent or dialect bias, Baxter says. Emerging best-practices for AI ethics demands that practitioners devise ways to detect specific types of bias that could impact their particular AI system, and to take steps to counter those biases.
“What type of bias did you look for? How did you measure it?” Baxter tells Datanami. “And then what was the score? What is the actual safe or acceptable threshold of bias for you to say this is good enough to be released in the world?”
Baxter’s is a more nuanced, and practical, view of AI ethics than one might get from textbooks (if there are any on the topic yet). She seems to recognize that you should accept from the outset that bias is everywhere in human society, and that it can never be fully eradicated. But we can hopefully eliminate the worst type of biases and still enable companies and their customers to reap the rewards that AI promises in the first place.
“You often hear people say, Oh we should follow the Hippocratic Oath that says do no harm,” Baxter says. “Well, that’s not actually the true application in medical or pharmaceutical industry, because if you said ‘no harm,’ there would be no medical treatment. You could never do surgery because you’re doing harm to the body when you’re cutting the body open. But the benefits outweigh the risks of doing nothing.”
There are ethical pitfalls everywhere. For example, it’s not just bad form to make business decisions based on the race or ethnicity of somebody–it’s also illegal. But the paradox is, unless you collect data about race or ethnicity, you don’t know if those factors are sneaking into the model somehow, perhaps through a proxy like ZIP Codes.
“You want to be able to run a story and see, are the outcomes different based on what someone’s races is, or based on what someone’s gender is?” Baxter says. “If it is, that’s a real problem. If you just say ‘No, I don’t even want to look at race, I’m just going to completely exclude that,’ then it’s very difficult to create fairness through unawareness.”
‘Sea of Vagueness’
The challenge is that this is all fairly new, and nobody has a solid roadmap to follow. Salesforce is working to build processes in Einstein Discovery to help its customers model data without incorporating negative bias, but even Salesforce is flying blind to a certain extent.
The lack of established standards and regulations is the biggest challenge in AI ethics, Baxter says. “Everyone is working in kind of a sea of vagueness,” she says.
She sees similarities to how the cybersecurity field developed in the 1980s. There was no security at first, and we all got hit by malware and viruses. That ultimately prompted the creation of a new discipline with new standards to guide its development. That process took years, and it will take years to hash out standards for AI ethics, she says.
“It’s a game of whack a mole in security. I think it’s going to be similar to AI,” she says. “We’re in this period right now where we’re developing standards, were developing regulations and it will never be a solved problem. AI will continue evolving, and when it does, new risks will emerge and so we will always be in a practice. It will never be a solved problem, but [we’ll continue] learning and iterating. So I do think we can get there. We’re just in an uncomfortable place right now because we don’t have it.”
AI ethics is a new discipline, so don’t expect perfection overnight. A little bit of failure isn’t the end of the world, but being open enough to discuss failures is a virtue. That can be tough to do in today’s volatile public environment, but it’s a critical ingredient to make progress, BCG’s Mills says.
“What I try to tell people is no one has all the answers. It’s a new area. Everyone is collectively learning,” he says. “The best thing you can do is be open and transparent about it. I think customers appreciate that, particular if you take the stand of, ‘We don’t have all the answers. Here are the things we’re doing. We might get it wrong sometimes, but we’ll be honest with you about what we’re doing.’ But I think we’re just not there yet. People are hesitant to have that dialog.”
Related Items:
Looking For An AI Ethicist? Good Luck
Governance, Privacy, and Ethics at the Forefront of Data in 2021
AI Ethics Still In Its Infancy