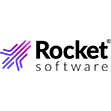
New AI Model From Facebook, Michigan State Detects & Attributes Deepfakes
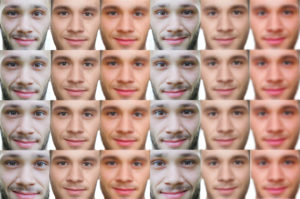
Deepfake technology allows for the easy digital transplant or manipulation of real faces, enabling users to depict, for instance, politicians saying things they never said. For many AI researchers – and many national security experts – the rapidly increasing accessibility and realism of this technology spells trouble for the near future. Now, researchers from Facebook AI and Michigan State University have teamed up to develop a new method for detecting and attributing deepfakes using reverse engineering.
“In some cases, humans can no longer easily tell some of them apart from genuine images,” wrote Xi Yin and Tal Hassner, research scientists at Facebook AI, in a blog post. “Although detecting deepfakes remains a compelling challenge, their increasing sophistication opens up more potential lines of inquiry, such as: What happens when deepfakes are produced not just for amusement and awe, but for malicious intent on a grand scale?”
Currently, deepfake detection models primarily identify whether or not an image is a deepfake or identify whether or not an image was created by one of the deepfake models used to train the attribution model. But, the authors note, deepfake models used for training are not the only ones that could be encountered in the wild, leaving an important gap in this attribution process.
This new model is different.
“Our reverse engineering method relies on uncovering the unique patterns behind the AI model used to generate a single deepfake image,” the researchers wrote. “We begin with image attribution and then work on discovering properties of the model that was used to generate the image. By generalizing image attribution to open-set recognition, we can infer more information about the generative model used to create a deepfake that goes beyond recognizing that it has not been seen before. And by tracing similarities among patterns of a collection of deepfakes, we could also tell whether a series of images originated from a single source.”
The researchers used fake faces (generated at MSU) to train the model, and MSU plans to open-source the dataset, code and trained models to facilitate further research. Since this type of model is new to the deepfake detection world, the researchers are as-yet unable to compare its results against a baseline. However, the researchers see wide applications for their work in the real world.
“This ability to detect which deepfakes have been generated from the same AI model can be useful for uncovering instances of coordinated disinformation or other malicious attacks launched using deepfakes,” they wrote. “This work will give researchers and practitioners tools to better investigate incidents of coordinated disinformation using deepfakes, as well as open up new directions for future research.”
To read the full blog from Facebook AI, click here.