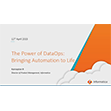
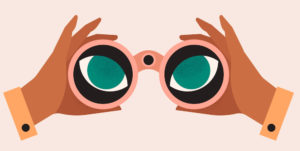
Data observability has emerged as one of the hottest sectors in the big data market, thanks to its focus on fixing broken data pipelines. One of the hottest players in the field is Monte Carlo, which this week announced a Series D round of funding worth $135 million, at a $1.6 billion valuation.
As companies look to data for competitive advantages, they’re finding that the costs of data quality problems continues to grow too. Gartner estimated that the average customer loses nearly $13 million per year due to data downtime and data quality problems. This is the area that Monte Carlo is addressing with its data observability offering.
Barr Moses and Lior Gavish co-founded Monte Carlo three years ago with the goal of developing tools to help companies detect problems in their ETL data pipelines and even take steps to automatically fix some of them. While ETL and ELT is viewed by some as a legacy approach to moving data, they continue to be the workhorse mechanisms for moving large amounts of data from on prem systems to the cloud, and everywhere in between.
The San Francisco company borrowed from concepts popular in the SRE and DevOps space to help address the problem of bad data flowing through data pipelines. By using connectors to take read-only copies of data directly from pipelines and machine learning techniques to spot anomalies in patterns, Monte Carlo is able to continuously monitor for common data problems, and send alerts to engineers when they are detected.
Monte Carlo looks for problems that can crop up across five main areas, including the freshness of data; its volume or completeness; whether the distribution of values is changing at the field level; whether data tables or schemas are shifting; and changes to data lineage. These are the companies five pillars of observability, which the company shared with Datanami in 2021.
There are a huge number of root causes to data issues, which isn’t Monte Carlo’s domain. (After all, if you have figured out a foolproof way to prevent humans from making data-entry mistakes, there are some folks on Sand Hill Road who would like a word).
Instead, Monte Carlo mainly looks to flag bad data as quickly as possible before it streams into downstream systems, including data warehouses and AI training systems. However, there are a handful of issues that Monte Carlo is looking to take immediate action on. Last month, the company launched Circuit Breakers to enable the company’s software to immediately end the flow of data in a data pipeline when one of these high-cost data errors, such as faulty data in a financial transaction, is detected.
The market need for data observability is growing quickly. For example, AutoTrader UK, uses Monte Carlo to keep a watchful eye on the proliferation of data models in its data analytics estate. While the Looker analytics software has been beneficial in lowering the barrier to entry for data analytics at AutoTrader UK, it has also increased the possibility that data errors can sneak into production, hence the decision to bring Monte Carlo in to automatically monitor the situation.
Monte Carlo has grown quickly as the need for data observability has increased, and users become aware there are solutions. Monte Carlo, which claims to have hundreds of customers, grew from 20 employees to 120 since late 2020, a period that coincides with several rounds of venture funding. In addition to AutoTrader UK, the company boasts customers like JetBlue, CNN, and SoFi.
Cack Wilhelm, a general partner at late-stage venture capital firm IVP, which led Monte Carlo’s Series D, said the need for high quality data has never been higher.
“Monte Carlo is charting the path forward for the data observability category and setting a precedent for the future of the modern data stack,” Wilhelm said in a press release. “After talking to dozens of Monte Carlo’s customers, two things became crystal clear: they are building a truly incredible product with near-immediate time to value, and they have one of the best teams in data. I’m excited to partner with Barr, Lior, and the rest of Monte Carlo on their vision for data reliability.”
Accel, GGV Capital, Redpoint Ventures, ICONIQ Growth, Salesforce Ventures, and GIC Singapore also participatd in Monte Carlo’s Series D. The company’s funding now totals $236 million over the past 20 months.
Related Items:
The Rise and Fall of Data Governance (Again)
Monte Carlo Hits the Circuit Breaker on Bad Data
February 20, 2025
- HarperDB Named an IDC Innovator for Edge Inference Delivery
- EDB Postgres AI Outperforms Oracle, SQL Server, and MongoDB in New Benchmark
- CData and Ellie.ai Partner to Streamline Enterprise Data Modeling
- Privacera Aligns AI Governance with NIST Standards to Mitigate AI Risks
February 19, 2025
- AtScale and Snowflake Announce Integration with Cortex Analyst to Deliver Trustworthy Natural Language Queries
- Sawmills Exits Stealth with $10M to Tackle Skyrocketing Observability Costs Using AI
- Prophecy Finds GenAI Boosting Data Team Productivity by Up to 50%
- CTERA Enhances Cybersecurity and AI Capabilities in Record-Setting Year
- VAST Data Introduces Event Broker for AI-Driven Real-Time Streaming
- DiffusionData Announces Free Trial of Diffusion Cloud
- Cube Launches Cube Cloud for the Microsoft Enterprise
- VAST Data Adds Block Storage to Unify Enterprise AI and Hybrid Workloads
February 18, 2025
- Boomi Unveils Comprehensive API Management to Combat Sprawl and Power Agentic AI
- Grid Status Launches Power Market Datasets on Snowflake Marketplace
- Gartner Predicts 40% of AI Data Breaches Will Arise from Cross-Border GenAI Misuse by 2027
- Fortanix Releases 2025 State of Data Security in GenAI Report
- Vultr Announces Availability of AMD Instinct MI325X GPUs to Power Enterprise AI
- SiMa.ai Advances Edge AI with Efficient Implementation of DeepSeek R1 Model
February 14, 2025
- OpenTelemetry Is Too Complicated, VictoriaMetrics Says
- What Are Reasoning Models and Why You Should Care
- Three Ways Data Products Empower Internal Users
- Keeping Data Private and Secure with Agentic AI
- Memgraph Bolsters AI Development with GraphRAG Support
- Three Data Challenges Leaders Need To Overcome to Successfully Implement AI
- Top-Down or Bottom-Up Data Model Design: Which is Best?
- PayPal Feeds the DL Beast with Huge Vault of Fraud Data
- What Leonardo DaVinci Teaches Us About Data Management
- Inside Nvidia’s New Desktop AI Box, ‘Project DIGITS’
- More Features…
- Meet MATA, an AI Research Assistant for Scientific Data
- AI Agent Claims 80% Reduction in Time to Complete Data Tasks
- DataRobot Expands AI Capabilities with Agnostiq Acquisition
- Snowflake Unleashes AI Agents to Unlock Enterprise Data
- Collibra Bolsters Position in Fast-Moving AI Governance Field
- Microsoft Open Sources Code Behind PostgreSQL-Based MongoDB Clone
- AI Making Data Analyst Job More Strategic, Alteryx Says
- EDB Says It Tops Oracle, Other Databases in Benchmarks
- Observo AI Raises $15M for Agentic AI-Powered Data Pipelines
- Anaconda’s Commercial Fee Is Paying Off, CEO Says
- More News In Brief…
- Informatica Reveals Surge in GenAI Investments as Nearly All Data Leaders Race Ahead
- Gartner Predicts 40% of Generative AI Solutions Will Be Multimodal By 2027
- PEAK:AIO Powers AI Data for University of Strathclyde’s MediForge Hub
- DataRobot Acquires Agnostiq to Accelerate Agentic AI Application Development
- Cloudera Welcomes Tom Brady as Keynote Speaker at ELEVATE26
- TigerGraph Launches Savanna Cloud Platform to Scale Graph Analytics for AI
- EY and Microsoft Unveil AI Skills Passport to Bridge Workforce AI Training Gap
- Alluxio Enhances Enterprise AI with Version 3.5 for Faster Model Training
- DeepSeek-R1 models now available on AWS
- Lightning AI Brings DeepSeek to Private Enterprise Clouds with AI Hub
- More This Just In…