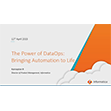
A New Era of Natural Language Search Emerges for the Enterprise
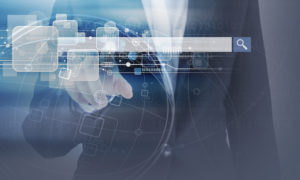
(ArtBackground/Shutterstock)
Large language models and their applications are suddenly a hot topic due to the popular release of OpenAI’s ChatGPT and the subsequent search engine wars between Google and Microsoft. ChatGPT and similar systems are revitalizing our idea of what the search experience can be. Instead of relying on specific keywords or complex search query syntax, users can now interact with search engines using human-like language.
Question answering systems are one capability of natural language processing, a set of language capabilities that LLMs enable, but QA systems have not always been a popular use case. Ryan Welsh, CEO of an NLP search company called Kyndi, recalls facing difficulties when explaining his company’s focus on NLP for search: “I remember raising money three years ago and everyone was like, ‘Hey, that’s cool, you’re an NLP, but this search thing is not a use case.’”
Welsh says this reaction has completely changed thanks to how many people are aware of natural language capabilities because of ChatGPT: “I feel like ChatGPT has done a decade’s worth of category evangelism in 90-120 days,” he said in an interview with Datanami.
Now, billions are being invested in next-generation search tech. There is suddenly a real market demand for QA systems that can give fast and accurate answers to questions asked by stakeholders or external customers visiting a company’s website or knowledge portal, as well as for internal employees searching company documents.
However, these current chatbot technologies are not meeting enterprise demands, Welsh says, noting that explainability, which is key for end-user trust, is often lacking. Enterprise requirements for a large language model system dictate that generated answers are accurate and trustworthy versus filled with hallucinations from training data sourced from web content, a problem that the large, mainstream models like ChatGPT face. Due to the statistical nature of their underlying technology, chatbots can hallucinate incorrect information, as they do not actually understand the language but are simply predicting the next best word. Often, the training data is so broad that explaining how a chatbot arrived at the answer it gave is nearly impossible.
This “black box” approach to AI with its lack of explainability simply will not fly for many enterprise use cases. Welsh gives the example of a pharmaceutical company that is delivering answers to a healthcare provider or a patient who visits its drug website. The company is required to know and explain each search result that could be given to those asking questions. So, despite the recent spike in demand for systems like ChatGPT, adapting them for these stringent enterprise requirements is not an easy task, and this demand is often unmet, according to Welsh.
Welsh says his company has stayed focused on these enterprise requirements over the years, learning from experience and direct interaction with customers. Kyndi was founded in 2014 by Welsh, AI expert Arun Majumbar, and computer scientist John Sowa, an expert in knowledge graphs who introduced a specific type called conceptual graphs at IBM in 1976.
Kyndi’s natural language search application builds on both knowledge graph and LLM breakthroughs by employing neuro-symbolic AI, a semantic approach that complements statistical machine learning techniques. Instead of just predicting the next most-likely word in text, the system creates symbolic representations of language, leveraging vector and knowledge graph technologies to map the relationships between data. This allows the system to understand the true intent behind an end-user question, helping to find context-specific answers while differentiating between common synonyms, semantically equivalent words, acronyms, and misspellings.
This technology needs little training data to be effective, which can alleviate bottlenecks caused by a lack of labeled data and AI expertise. The high costs associated with data labeling make training and fine-tuning LLMs prohibitively expensive for many enterprises. This ease of tuning is another differentiating factor of Kyndi’s neuro-symbolic approach. Welsh says many enterprise customers have been burned by slow AI deployments. He describes a large pharmaceutical company that, before working with Kyndi, had been tuning an LLM using six machine learning engineers and data scientists for over six months. Welsh says Kyndi was able to train and tune their model in one day with just the help of a business analyst. In several other cases, Kyndi was able to take AI projects through demo, sandbox validation, and deployment within two weeks.
One Kyndi customer is the U.S. Air Force which uses the company’s answer engine system to search its flight mission reports and human intelligence data that spans decades to now. Welsh says the system has been transformational for the military branch, as the self-service Kyndi platform allows intelligence analysts and leaders to ask questions like “Was there an intervention anywhere in the world today?” (meaning a non-conflict interaction with another country’s airspace or the like) and get instant answers. Welsh says successful, large-scale use cases like this point to the future of search.
“I think every search bar, and every chat interface, in every enterprise in the world, is going to have an answer engine behind it at some point in the next 10 years. And it’s going to be the biggest kind of transition that we’ve seen in enterprise software,” Welsh says, comparing this moment to the transition from on-prem to cloud. “I don’t think there’s any vendor that is positioned presently to dominate that market.”
Welsh predicts the companies that will win during this new era of the enterprise search space are those that had the foresight to have a product on the market now, and though the competition is currently heating up, some of these newer companies are already behind the curve. He estimates they have about 2-3 years and $30 million worth of building to do before they have a product that can be deployed by a military agency or pharma company.
Welsh says Kyndi has found success through its differentiating factors and is checking the boxes for many enterprise customers: “When we come through, and we talk to customers, and they say, ‘Hey, we need accurate answers from trusted enterprise content, it has to be explainable, and it has to be easy to run in an enterprise environment, we’re going check . . . check . . . check.”
Related Items:
Companions, Not Replacements: Chatbots Will Not Succeed Search Engines Just Yet
Kyndi Bolsters NLP with Search-as-a-Service
Are Neural Nets the Next Big Thing in Search?