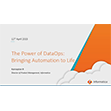
The Face of Data Bias
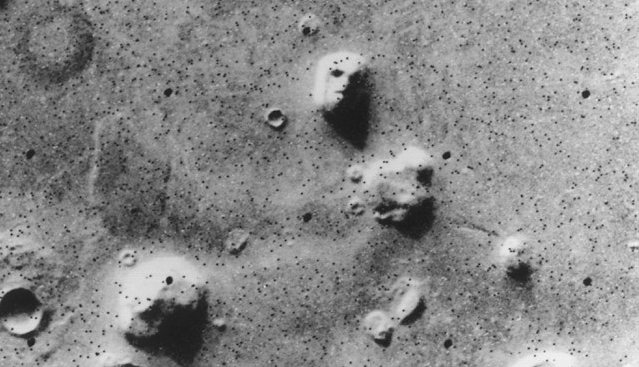
Source: NASA/JPL
What do you see when you look at this photo?
Most will see a picture of a face. A face on a planet other than Earth. Shocking, right? An extraterrestrial object resembling a human face.
Below is the same geological feature, taken 21 years later. Still see a face, or do you now see a mountain?
This image is one of the more famous examples of pareidolia. Pareidolia is the tendency for us to see patterns in nebulous images or surroundings and attribute meaning, in this case, a face where there is none. Our brains have this hard-wired into it because of the importance of faces in our evolutionary history.
You have seen this elsewhere, in curtains, clothing, fabrics – see a face where none exists. It is a form of bias. It comes from a lack of data from the input. Missing fragments of information that we then, instinctually, fill in so that we can “understand” what we are seeing.
Interestingly, scientists viewing that first image knew that data was missing in the image they received, so-called bit errors. They were caused by problems in transmission of the photographic data from Mars to Earth. These bit errors even caused some of the visual elements that made the feature resemble a face.
These errors led to some amusing reactions, even whole books about structures on Mars. And it was not the first time our celestial neighbor had some wild claims about what might exist on the surface. In the latter half of the 19th century, when astronomers looked through their telescopes, they saw lines on the surface as canals.
This comes from a lack of data, and the intrinsically human trait to find patterns in the world, and beyond, to make sense of what we see.
The human brain does the same. When reading, the reader does not need the whole word in the correct order. It is possible to read lietrautre even with the letters in the wrong place if the first and last are correct. Do not believe me? Go back and look at the word literature.
Why is this important, and what does it have to do with data bias and today’s businesses? Data bias, whether it is racial, ethnic, or stems from another form, exists in all lives. It can be hardwired from our nature, as above, or nurtured throughout our lives. As a result, it can make its way into technology as well.
Data bias can and does affect how we understand our business or organization and leads to blind spots in many places. Whether it is research, safety, or population data, missing criminal statistics, or in the worst-case deliberate omissions, data can harm both the business and end users. Injustices, employment, financial inequalities, mischaracterization, medical malpractice, the list goes on.
More concerning is the scale of data bias’s impact. With machine learning (ML), Artificial Intelligence (AI), and other data-related technologies being used at scale, with 100s of millions of users worldwide, the impact that errors in the data can cause are wide-ranging – up to and including, in rare circumstances, life-threatening.
Simply recognizing this does not fix the issue. A recent study conducted by Insight Avenue showed that while 78% of business and IT leaders believe data bias will become a bigger problem, 77% of respondents acknowledge that they need to do more to understand and address bias. Only a mere 13% are actively working to confront and end data bias through an ongoing evaluation process.
Businesses and organizations must act. They need to meticulously assess their data sets, identify bias in all its forms, and respond appropriately to remove, update, amend, or secure the data to gain the most comprehensive understanding of their business and the world they operate in. Equally crucial is the evaluation of the technology used. Data platforms that can capture both structured and unstructured data while establishing robust guardrails to govern and protect the data are vital.
Additionally, using technology to supply human context, meaning, and insights at machine scale is essential. Exploring connections within the data and applying metadata to its source can contribute to a more accurate representation. These efforts should precede and follow the data’s use in any system, especially in ML and AI systems, where the scale of bias amplification can lead to inaccurate conclusions.
Businesses need to trust the data they use, the data-driven decisions made, and the actions taken to ensure the outcomes desired are correct. Failure to tackle data bias adequately could result in a loss of trust from end-users, potentially affecting the success and sustainability of enterprises overall.
About the author: Philip Miller is a customer success manager for Progress and was named a Top Influencer in Onalytica’s Who’s Who in Data Management. Outside of work, he’s a father to two daughters, a fan of dogs and an avid learner, trying to learn something new every day.
Related Items:
Will Analytics Help Reduce Our Biases or Amplify Them?
Three Ways Biased Data Can Ruin Your ML Models
Beware of Bias in Big Data, Feds Warn