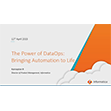
KNIME Releases a State of Data Science and Machine Learning Survey
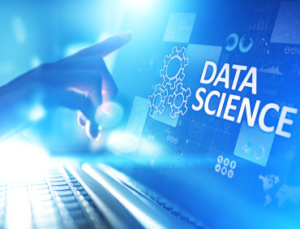
KNIME, a data science company focused on making analytics accessible to all, in collaboration with Enterprise Strategy Group (ESG) released a survey on the state of data science and machine learning. ESG is a research, validation, and strategy firm that provides market intelligence. The survey highlights how organizations are prioritizing, investing, and operationalizing data science, artificial intelligence and machine learning. The findings also uncover the most pressing challenges in the data science processes and how to address them.
Organizations are facing challenges in machine learning projects. For example, some organizations are struggling to successfully integrate machine learning models into their software development lifecycle. The handling of large and complex data sets, managing specialized hardware, the gap between different skill sets, and ensuring availability, scalability, and security in production collectively add to the challenge.
Such challenges highlight the need for clear data science and machine learning strategies. As part of these strategies, an increasing number of organizations are recognizing the importance of taking a standardized and structured approach to developing, deploying, and maintaining ML Models.
To gain further insights into these trends, ESG surveyed 366 professionals at different organizations across the U.S. and Canada. These surveyed professionals were involved in data science and machine learning technologies and processes, including strategizing, building, and managing such technologies.
The objective of the study was to identify investment plans, objectives, and challenges of data science and machine learning. This included the current state of operationalizing AI through MLOps and how organizations are prioritizing solutions to best help them succeed.
Here are some of the key findings of the data science and machine learning survey.
Challenges Loom Large
Nearly a third of organizations (27 percent) say a lack of skilled talent stands in the way of developing and implementing data science projects. The other key challenges include insufficient integration with existing systems (25 percent) and limited budget and resources (23 percent).
Around 35 percent of companies are finding it difficult to manage multiple environments for ML technologies. Other challenges for ML included difficulty ensuring compliance with corporate governance policies (33 percent) and difficulty detecting and responding to data drift (33 percent).
Primary Business Objectives Point Inward
Improving operational efficiency remains a top priority of organizations, who are realizing that once operations are at an optimal level they can focus on other business imperatives to help build a foundation for sustainable growth in the increasingly data-driven business world.
Based on the survey results, the primary business objectives driving data science and machine learning initiatives included improving operational efficiency (66 percent), improving product development and innovation (60 percent), and enhancing customer experience or improving customer satisfaction (52 percent). Budgets Are on the Rise
Around 92% of organizations had a year-to-year increase in budget allocations for data science and machine learning. Nearly one in four companies planned to invest at least $1 million in technology, processes, or people that are associated with data science and machine learning.
The rise in budgets highlights the understanding that data science not only enhances operational efficiency, but also enables better predictive analytics, informed decision-making, and innovative product development.
Focus Sharpens on Improving Early and Late Stages of Data Science Lifecycle
The top factors when considering purchases to support data science initiatives included integration with existing systems (34 percent) and ease of implementation and deployment (33 percent). Simplifying implementation and deployment highlights the need for companies to improve the time between data generation and data insights.
Around 26% of organizations valued compatibility with open-source technologies as an important factor when making purchases to support data science initiatives, likely foreshadowing a larger open-source deployment trend moving forward.
Stakeholder Involvement Across the Data Science Lifecycle
Based on the results of this survey, non-data stakeholders play a key role across the data science lifecycle from data collection to model management. This is why 92% of respondents rate the experience of non-data science professionals (business stakeholders) being involved in data science initiatives and working with data science teams as positive, if not very positive.
The survey also highlighted the employees’ drivers to improve skills in data science and machine learning. The top three drivers included career advancement opportunities (52 percent), keeping up with industry needs (50 percent), and job security (45 percent).
Related Items
KNIME Releases New User Experience and AI Assistant
Comet Releases MLOps Industry Report | 2023 Machine Learning Practitioner Survey
Dataiku and Databricks Survey Reveals the Power of AI: Over 70% of Professionals See Positive ROI