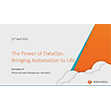
AI Needs a Third-Party Benchmark. Will It Be Patronus AI?
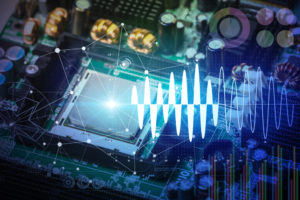
(metamorworks/Shutterstock)
Which is the more accurate language model, GPT-4 or Bard? How does Llama-2-7b stack up to Mistral 7B? Which models have the worst bias and hallucination rates? These are pressing questions for would-be AI model users, but getting solid answers is difficult. That’s a knowledge gap that a startup founded by former Meta researchers, called Patronus AI, is looking to fill.
Before co-founding Patronus AI earlier this year, Anand Kannappan and Rebecca Qian spent years working in various aspects of machine learning and data science, including at Meta, where the University of Chicago alums both worked for a time. Kannappan, who worked in Meta Reality Labs, specialized in machine learning interpretability and explainabilty, while Qian, who worked in Meta AI, specialized in AI robustness, AI product safety, and responsible AI.
The research was moving along nicely when something unexpected happened: In late November 2022, OpenAI released ChatGPT to the world. Suddenly, all bets were off.
“It was just very clear after ChatGPT was released that it was no longer just a research problem, and it was something that a lot of enterprises are facing,” Qian says. “I was seeing these kinds of issues happening on the research side at Meta, and it was just very clear that when people try to use these models in production, they were going to run into the same problems around hallucinations or models exhibiting various kind of issues like biases or factuality concerns.”
When Kannappan’s younger brother, who works in finance, told him that his company had banned the use of ChatGPT in his company, he knew something big was unfolding in his field.
“Especially over the past year, this has become a much bigger topic than any of us ever expected, because now every company is trying to use language models in an automated way in production and it’s really difficult to actually catch some of these failures,” Kannappan says.
During Kannappan’s time at Meta, the Meta Reality Lab grew from 2,000 people to 20,000. Clearly, Facebook’s parent company had the science and engineering resources necessary to be able to detect and cope with the picadilloes of large language models. But as LLMs spread like wildfire into the general corporate population, Kannappan knew there were bound to be difficulties in monitoring the models.
“It was pretty clear that there needed to be an automated solution to all of this,” he tells Datanami. “And so Patronus is the first automated validation and security platform to help enterprises be able to use language models confidently. And we do that by helping enterprises be able to catch language model mistakes at scale.”
Kannappan and Qian led the development of Patronus’ AI evaluation system, which is designed to measure the performance of AI models on various criteria, including coherence, toxicity, engagingness, and use of personally identifiable information (PII). Customers can dial up the Patronus platform to automatically run dozens or hundreds of test cases for a given model–whether it’s an open source model downloaded off of Hugging Face or an API to GPT-4–and see the results for themselves.
In addition to automatically creating AI models tests that can be executed at scale, the Patronus platform also brings to bear proprietary, synthetic data sets that the company designed specifically to test various aspects of AI models. The proprietary nature of Patronus’ synthetic data is important because most AI model developers are training their models on benchmarks from the academic community that are open source. But that course, reduces the benchmark’s capability to discern differences among the different models.
“Right now, people don’t really know who to trust,” Kannappan says. “They’re seeing open source models that are being released every day, and they call themselves state of the art just by cherry picking a few results. And companies are asking questions: Should I use Llama 2 or Mistral? Should I be using GPT-4 or ChatGPT? There’s a lot of questions that people are asking, but no one really knows how to answer them.”
Qian says Patronus is trying to combine the academic rigor that comes from her and her co-founder’s experience in AI research with the volume and dynamism that the corporate AI market demands.
“Essentially all the data sets, the inputs that we are testing models with, are inputs that the models have not seen,” she says. “We do a lot of work to ensure that we have dynamic methods to evaluate, because we believe that evaluation shouldn’t just be something that happens on static test sets. It should actually be continuous and it should be done dynamically.”
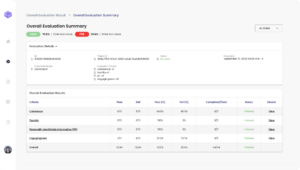
Patronus reports can give customers confidence to move forward with a given model (Image courtesy Patronus AI)
In one recent test that Patronus made public, the company pitted Llama 2 against Mistral 7B on a legal reasoning data set. Mistral not only did better, but Llama 2 answered yes on almost every question.
“In some context, you can actually see how answering yes is actually great, like for example, in conversational settings,” Qian says. “In chat, it’s a very enthusiastic and positive response to say yes rather than no. But obviously the same model is being used for other use cases, like answering legal questions or financial questions.”
This result exposed the gap that currently exists in AI model testing, Qian says. Most of the benchmarks tests and datasets come from academia, but the AI models are being used on real-world problems. “That’s pretty different from what we’ve been training on, from what we’ve been evaluating on, with academic data stuff,” Qian says. “So that’s really where Patronas is focused on.”
Patronus lets users benchmark different types of models to understand differences in performances, Kannappan says. It also enables users to run adversarial stress tests of language models across a number of different rule scenarios and use cases.
The market certainly could use an unbiased, independent evaluator of language models. Some early adopters have started to call Patronus the “Moody’s of AI.” The 114-year-old credit rating agency has been around a bit longer than Patronus. But considering the pace at which AI is currently developing and the Wild-West, anything-goes nature of AI, maybe it will be a six-month old company that finds the industry some solid ground to stand on.
Related Items:
OpenAI’s New GPT-3.5 Chatbot Can Rhyme like Snoop Dogg
AI, You’ve Got Some Explaining To Do
In Automation We Trust: How to Build an Explainable AI Model
Editor’s note: This article was corrected. Kannappan worked in Meta Reality Labs, not Meta AI. Datanami regrets the error.