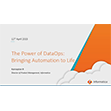
Leveraging GenAI and LLMs in Financial Services
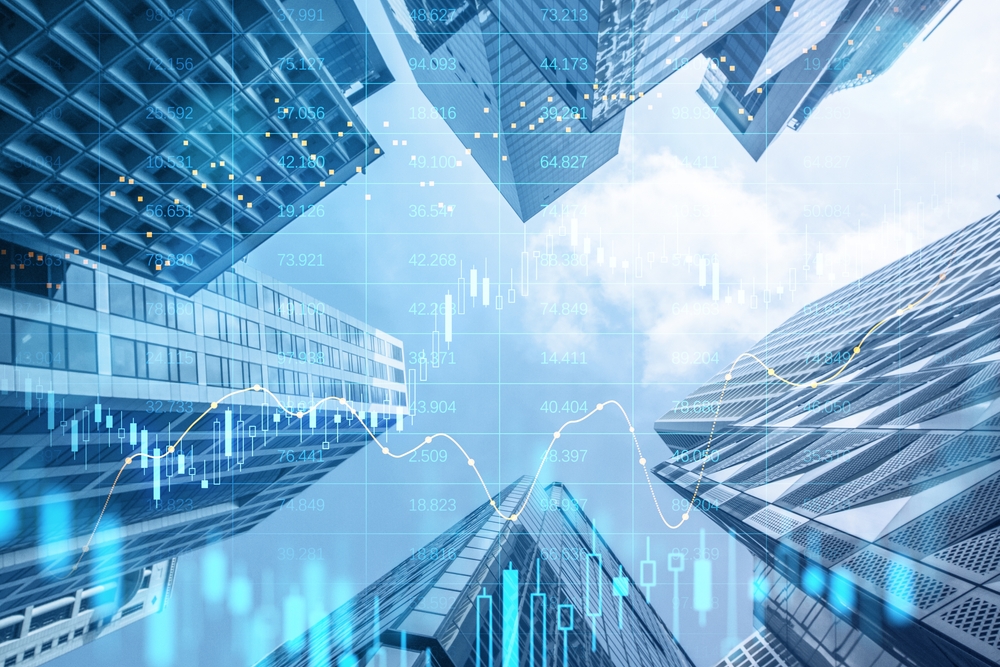
(Golden Dayz/Shutterstock)
Data and large language models (LLMs) can save banks and other financial services millions by enhancing automation, efficiency, accuracy, and more. McKinsey reports that the productivity lift from generative AI can lead to an increase of 3-5% of annual revenue in the banking sector, which is equivalent to $200 billion to $340 billion of additional annual revenue.
Given the large amount and variety of data that is available in the financial industry, LLMs can bring significant value-add to financial services businesses. Below are just a few examples of how Gen AI and LLMs can support financial services.
Fraud Prevention: Generative AI is at the forefront of developing cutting-edge fraud detection mechanisms. By analyzing vast data pools, it can discern intricate patterns and irregularities, offering a more proactive approach. Traditional systems, often overwhelmed by the sheer volume of data, might produce false positives. Generative AI, in contrast, continuously refines its understanding, reducing errors and ensuring more secure financial transactions.
Compliance: Anti-Money Laundering (AML) regulations are critical in maintaining the integrity of financial systems. Generative AI simplifies compliance by sifting through intricate transactional data to pinpoint suspicious activities. This not only ensures financial institutions adhere to global standards but also significantly reduces the chances of false positives, streamlining operations. A Generative AI model can help create more efficient screening and faster document analysis, along with monitoring and reporting tasks.
Data-driven decision-making: Given the potential to work with unstructured text data, LLMs are able to draw insights from data sources such as news reports, social media content, and publications. This allows companies in the financial industry to draw from underutilized sources.
Customer interaction and support: LLMs have boosted the capabilities and expectations we have around chatbots and virtual assistants. LLM-powered chatbots such as ChatGPT have shown an immense capacity for human-like communication experiences. Incorporating these chatbots into financial customer support services will improve the efficiency and the nature of customer interactions. For instance, a virtual personal adviser who can provide tailored insight into investments or personal financial management can be extremely well-received by customers.
Business innovation and efficiency: We have recently seen a surge of LLM-based add-ons for existing tools and technologies. For instance, natural language-based instructions, programming assistants, and writing assistants are becoming extremely common. These LLM-based functionalities can bring about significant innovation and efficiency to the finance industry.
What Are the Challenges?
Training LLMs with financial data: LLMs are currently trained on the Internet. Financial services use cases will require fine-tuning these models with use case-specific financial data. New entrants will probably start refining their models with public company financials, regulatory papers, and other sources of easily accessible public financial data before eventually using their own data as they collect it over time.
Existing players, like banks or large platforms with financial services operations, can leverage their existing and proprietary data, potentially giving them an initial advantage. Existing financial services companies, however, tend to be overly conservative when it comes to embracing large platform shifts. This likely gives the competitive edge to unencumbered new entrants.
Model output accuracy: Given the impact the answer to a financial question can have on individuals, companies, and society, these new AI models need to be as accurate as possible. They can’t hallucinate or make up wrong but confident-sounding answers to critical questions about one’s taxes or financial health, and they need to be far more accurate than the approximate answers for popular culture queries or generic high school essays. To start, there will often be a human in the loop as a final verification for an AI-generated answer.
Bias Amplification: AI models, as sophisticated as they are, still rely on human-generated training data. This data, with its inherent biases—whether intentional or not—can lead to skewed results. For instance, if a particular demographic is underrepresented in the training set, the AI’s subsequent outputs could perpetuate this oversight. In a sector like finance, where equity and fairness are paramount, such biases could lead to grave consequences. Financial leaders need to be proactive in identifying these biases and ensuring their datasets are as comprehensive and representative as possible.
Data Privacy & Compliance: Protecting sensitive customer data remains a significant concern with generative AI applications. Ensuring the system adheres to global standards like the General Data Protection Regulation (GDPR) and the California Consumer Privacy Act (CCPA) is crucial. AI may not inherently know or respect these boundaries, so its use must be moderated with stringent data protection guidelines, particularly in the financial sector where confidentiality is paramount.
Quality of Input Data: Generative AI is only as good as the data fed to it. Inaccurate or incomplete data can inadvertently lead to subpar financial advice or decisions.
LLMs will increase efficiency by automating and streamlining a variety of tasks. It will enable companies to make better sense of data, particularly unstructured text data, thereby allowing for more informed decision-making. With higher natural language processing capabilities led by LLMs, customer-oriented tools such as chatbots will be more capable of taking on a larger portion of customer support, as well as providing improved support services. This will improve the quality of customer experience while freeing up valuable human time and capacity to engage in more value-generating tasks.
About the Author: Namrata Ganatra, Chief Product and Technology Officer of Pipe has over a decade of experience and a proven track record of leading successful initiatives and contributing to the growth of several notable companies in fintech, AI, and crypto. Namrata’s recent experience includes leading product and engineering at Autograph, a leading NFT platform. She also founded a Generative AI startup that helped SMBs grow multi-channel e-commerce sales, which was successfully acquired by Thrasio. Prior to that, Namrata held senior roles at Coinbase and Facebook, where she played a critical role in shaping payment strategy and scaling payment infrastructure to meet the needs of millions of users. Namrata is also an Angel Investor and Advisor at Distyl AI, which has partnered with OpenAI to build Generative AI Solutions for Enterprises.
Related Items:
How Large Language Models and Humans Can Make Strategic Decisions Together
GenAI Hype Bubble Refuses to Pop
LLMs Are the Dinosaur-Killing Meteor for Old BI, ThoughtSpot CEO Says