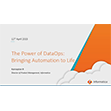
Bringing Data and ML to Value-Based Pricing
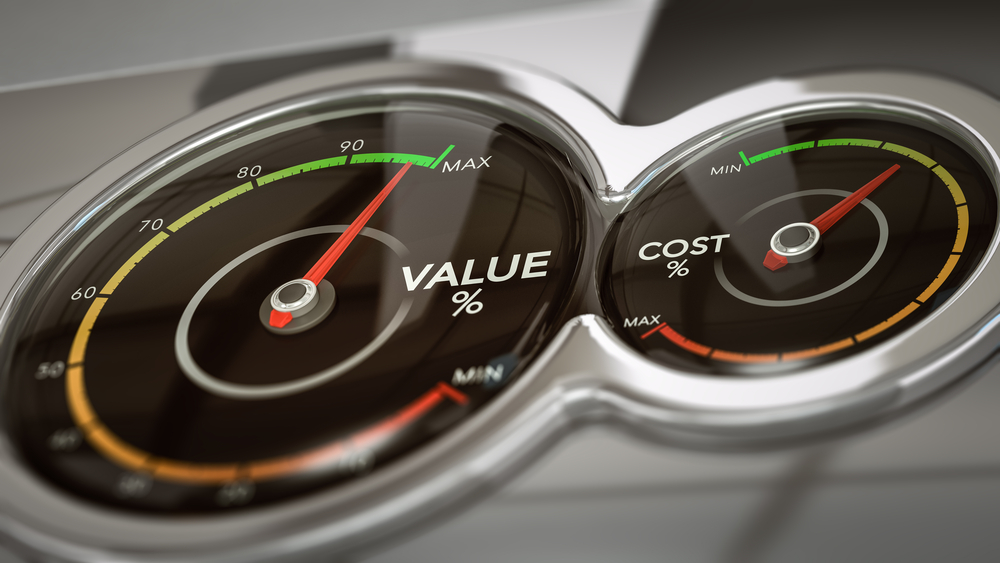
(Olivier Le Moal/Shutterstock)
Companies across many industries historically have used a cost-based approach to setting prices for goods and services. But many are starting to explore an entirely different approach called value-based pricing. When combined with data collection and machine learning algorithms, the value-based pricing approach can be very powerful.
With traditional cost-based pricing strategy, sellers determine the price a particular item by adding up the various costs they incurred (such as for manufacturing, distribution, transportation, marketing, etc.) and then apply a fixed markup. Cost-based pricing, which is sometimes called cost-plus pricing, is especially common in the consumer goods supply chain, where companies may tout their markups.
Value-based pricing takes an entirely different approach. Instead of an inward-facing strategy focused on costs and expected profit, a value-based pricing looks outward to the customer to determine what kind of value the customer will receive from the good or service.
According to Investopedia, value-based pricing is better geared toward more complex products and services, and can allow a seller to maximize the price at which they ultimately sell goods or services, while also helping to promote customer and brand loyalty.
“While value-based pricing is resource-intensive because it requires gathering and analyzing customer data, it can lead to advantages in sales, elevated price points and customer loyalty, and other benefits,” writes Andrew Bloomenthal in his Investopedia article.
One big proponent of value-based pricing is Fabrizio Fantini, the vice president of product strategy at ToolsGroup. Fantini, who wrote a doctoral thesis titled “Online algorithm for dynamic pricing” for his PhD in Applied Mathematics from ESCP Business School in Paris, France, helps companies implement sophisticated value-based pricing strategies around the world.
“Frankly it’s nothing complicated,” Fantini tells Datanami in a recent interview. “In a nutshell, it’s the idea that a good price is one that works for the intersection of you and your client. It’s a mindset more than an algorithm. Once you raise that mindset, it can be really simple.”
There’s no set formula for value-based pricing, and what determines that optimal price in a value-based pricing system can be different things. It could depend on the features or aspects of the product, or the particular season. People in different geographies value things differently. There are psychological aspects too, such as the reluctance that people demonstrate to breaking a $20 bill.
Value-based pricing also requires more work on the part of the seller. Not only must they analyze their own objectives, they must be willing to learn and re-learn lessons that the market is willing to teach them–if they are attuned to hear them.
“If you ask a manager what’s their objective, they’ll tell you they want more profit. Okay fine. We all agree. We’re all happy,” Fantini says. “It turns out that’s not actually what companies are there for. Of course profit is one of them, but they also want more cash, more revenue, more loyalty, better perception.”
Machine learning algorithms can be very helpful in implementing a value-based pricing scheme. According to Fantini, the signal required to build a value-based pricing system can be found in the mix of common sales data, including product, location, and people. This data will help a company begin to determine where the price points are where customers perceive they’re getting value from the product and where they’re not.
Succeeding with value based pricing is all about framing the question the question appropriately, being receptive to what the data is telling you, and understanding that the answers today will change as the world changes, Fantini said.
“You can only discover these things by being humble enough and learning that aggregated demands doesn’t necessarily function according to the rational scheme that you have in mind,” he says.
The open-ended nature of value-based pricing can lead to all sorts of data types being collected and analyzed. Humans have an infinite appetite for granularity, Fantini says. That may be intimidating at first. The good news is companies can get started without breaking the bank on a massive data collection effort.
“You don’t need that much data. That’s a counter-intuitive thing,” he says. “To begin, you may do with a surprisingly little amount of data, if you frame your questions right. Data and algorithms are important. I don’t want to discount that completely. But the truth is you actually can get away with surprisingly little amount of data, so long as you have a very good framework on top of it.”
It’s important to understand there is data granularity on the supply side, such as assessing the product mix across time and space, but there is also granularity on the demand side, such as how discounts, promotions, or weather drives people to buy. These variables need to be treated carefully, since comparing companies with different products and customers is fraught with danger.
When it comes to being data driven and using AI, being able to ask the right question of the data is much more valuable than having more data. “To do value-based pricing, you need to have a different logic. You need to be constantly adjusting your thinking based on what you’re finding out in the market, and that’s very hard,” Fantini says.
Success at value-based pricing does require good data and a good model. But machines don’t think in nuances, and so it’s more important to have somebody who can ask the right questions of the data–and to do so quickly before the market opportunity is gone, Fantini says.
“The human capability is where the gap is,” he says. “We’ve been trained in the wrong skill. The real skill is framing the problem. And machines are really stupid, so you really need to ask them simple, laser-targeted questions.”
Fantini likes the idea of an invisible hand guiding the market, helping buyers and sellers come together on a price that works for both them. AI can help that invisible hand work more efficiently by bring the seller to the price point where the customer experiences the greatest value.
“That’s basically a sustainable source of competitive advantage,” he says. “People who master that technique are smart about designing for price, designing for demand. They’re not just changing prices.”
Related Items:
Top 10 Ways AI Drives Price Optimization in Retail
The New Omnichannel Imperative: AI to the Rescue
Digital to Profit: When AI and Machine Learning Meet Pricing