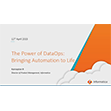
AWS Expands Sagemaker To Combine Data, Analytics, and AI Capabilities
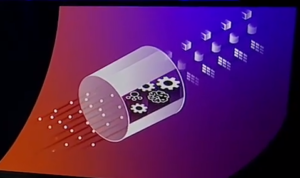
AWS SageMaker has long served as the go-to platform for managing the entire lifecycle of machine learning (ML) and GenAI models. It offers tools to build, train, and deploy these models. The platform is also used to access pre-trained models, build foundation models (FMs), and refine datasets.
However, there has been a growing need for additional tools to handle other aspects of the ML lifecycle, such as governance tools and automated validation. While various tools exist to address such needs, many of them operate outside the SageMaker ecosystem. This fragmentation often adds complexity, inefficiency, and increased overhead for users.
To address these challenges, AWS has introduced a comprehensive environment with its next-generation SageMaker features, announced at the re:Invent 2024 conference. The update is designed to offer a unified hub for data, analytics, and AI tools.
The introduction of the next-generation SageMaker comes at a time when there is a growing trend of enterprises using data in interconnected ways. This convergence of AI and analytics could help enable businesses to leverage their data for a range of functions, such as improving predictive maintenance and enhancing customer personalization.
“We are seeing a convergence of analytics and AI, with customers using data in increasingly interconnected ways—from historical analytics to ML model training and generative AI applications,” said Swami Sivasubramanian, vice president of Data and AI at AWS.
“To support these workloads, many customers already use combinations of our purpose-built analytics and ML tools, such as Amazon SageMaker—the de facto standard for working with data and building ML models—Amazon EMR, Amazon Redshift, Amazon S3 data lakes, and AWS Glue.
“The next generation of SageMaker brings together these capabilities—along with some exciting new features—to give customers all the tools they need for data processing, SQL analytics, ML model development and training, and generative AI, directly within SageMaker.”
The upgrade includes the SageMaker Unified Studio which provides a single data and AI development environment where users can find and access all of the data in their organization. This tool integrates key tools from AWS, such as Amazon Bedrock, making it easier for users to manage their data, develop ML models, and build GenAI applications.
AWS shared that NatWestGroup, a leading bank group in the UK, is set to use SageMaker Unified Studio across the organization to support various workloads, including data engineering and SQL analytics. AWS claims that this unified environment will help the bank reduce the time data users spend accessing analytics and AI capabilities by 50%.
As part of its ongoing efforts to enhance AI governance and enterprise security, AWS introduced the Catalog feature in SageMaker. This tool enables users to define and implement consistent access policies with granular controls. Built on Azure Datazone, Sagemaker Catalog helps safeguard AI models with toxicity detection, responsible AI policies, data classification, and guardrails.
A key upgrade to the platform is the introduction of the new SageMaker Lakehouse. It helps reduce data silos by enabling AI, ML, and analytical tools to query and analyze data across various storage systems throughout the organization. Additionally, the platform is compatible with Apache Iceberg open standards, allowing customers to work with their data efficiently for SQL analytics.
AWS shared that Roche, a Swiss pharmaceuticals and diagnostics company, anticipates a 40% reduction in data processing time using SageMaker Lakehouse to unify data from Redshift and Amazon S3 data lakes. This allows businesses to focus more on achieving their strategic goals and less on data management. Customers also get to use their preferred analytics and ML tools on their data, regardless of where the data is stored.
SageMaker Lakehouse supports Apache Iceberg, making it compatible with various AI, ML, and query tools that use the open standard. It also offers zero-ETL integrations for Amazon Aurora MySQL, PostgreSQL, RDS for MySQL, and DynamoDB, as well as popular SaaS applications like Zendesk and SAP.
These integrations allow businesses to efficiently access and analyze data without building complex data pipelines. This reflects AWS’s broader strategy to simplify data workflows for analytics and ML, creating a unified environment for data processing and insight generation.
“Organizations of all sizes and across industries, including Infosys, Intuit, and Woolworths, are already benefiting from AWS zero-ETL integrations to quickly and easily connect and analyze data without building and managing data pipelines,” AWS noted in a press release.
“With the zero-ETL integrations for SaaS applications, for example, online real estate platform Idealista will be able to simplify their data extraction and ingestion processes, eliminating the need for multiple pipelines to access data stored in third-party SaaS applications and freeing their data engineering team to focus on deriving actionable insights from data rather than building and managing infrastructure.”
SageMaker’s next-generation platform is already available, with the SageMaker Unified Studio currently in preview. While AWS has not provided a specific timeline, it mentioned that the SageMaker Unified Studio is expected to be generally available soon.
Related Items
AWS Bolsters GenAI Capabilities in SageMaker, Bedrock
AWS Takes On Google Spanner with Atomic Clock-Powered Distributed DBs
AWS Unveils Hosted Apache Iceberg Service on S3, New Metadata Management Layer