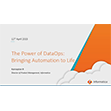
Beyond the Buzz: How Enterprises Can Leverage GenAI for Tangible Results
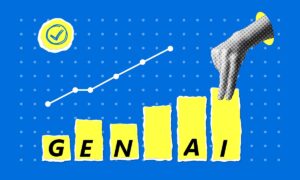
(hmorena/Shutterstock)
The public release of ChatGPT in November 2022 set off a wave of hype over generative AI (GenAI) unlike anything seen in the technology sector since the introduction of the public Internet. Now, almost two years later, it’s clear that generative AI isn’t the magic bullet many envisioned, and Gartner has declared that GenAI has officially entered the “trough of disillusionment. In a nutshell, GenAI’s whiz-bang appeal is waning as organizations struggle to achieve ROI and see value from it.
The problem is not GenAI as a technology. The hype was not, for the most part, overblown. GenAI’s uncanny ability to understand, summarize and produce text, audio, images, video and other content truly is a revolutionary, disruptive technology. GenAI can summarize documents that are hundreds of pages long in just a few minutes, understand requests in natural language, create workable low-level code in seconds, and provide results in essentially any format the user desires.
The Root Cause of GenAI Disillusionment
GenAI is far from being artificial general intelligence (AGI), which is the ultimate goal of many AI researchers and is the technical term for an AI that can perform essentially the same tasks as a human mind. As Incredible as it is, GenAI does have limitations, because it is built on a large language model (LLM). While It excels at parsing speech and text, it is not designed to crunch numbers and perform analysis. Even operations as simple as counting can be hit or miss, as anyone who has asked GenAI to provide text with a certain word count can verify. And, of course, there’s the well-known hallucination problem, where GenAI creates facts and references that don’t exist.
GenAI has entered the “trough of disillusionment” for a variety of reasons, including:
- A lack of internal AI expertise: Training, deploying and maintaining generative AI on your own is difficult even if an organization has the highly specialized skills required. Unfortunately, people with these skills are expensive to hire and in short supply.
- The scarcity of specialized hardware on which to run AI: Significant GenAI deployments often require high-performance chips such as GPUs. Like highly skilled AI specialists, this gear is scarce. Deployments on less performant hardware results in a poorly performing GenAI implementation.
- The cost of training up GenAI models: Training a large language model (LLM) requires expensive skills and gear, plus a ton of power. By 2027, AI is expected to consume 5% of the world’s electricity.
- Lack of guardrails and security: GenAI has a well-known problem with hallucinations, creating “facts” that don’t exist. Likewise, GenAI needs strong security to protect the sensitive data it will work with and produce, as well as guardrails and policies that guide use from a policy and ethical standpoint.
The trough of disillusionment isn’t permanent, however. GenAI is clearly a useful technology, and with creative thinking and the integration of GenAI with other technologies that would benefit from its ability to analyze, summarize and create content, enterprises can climb out of it. The key is to find valuable use cases that play to GenAI’s current strengths and, where GenAI has weaknesses, combine it with complimentary technologies, guardrails and security measures that can shore them up.
A Use Case that Delivers ROI
In the near term, GenAI has the enormous potential to create value as the ultimate interface for critical and complex applications. Just about any application can benefit from a more intuitive UI, and nothing is more intuitive than plain language. GenAI makes this possible, which is why vendors have begun integrating it into their products. So long as IT fully vets the vendor’s GenAI implementation for the application, the enterprise gains a lot of upside with very little downside.
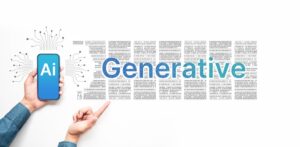
Incorporating GenAI’s language capabilities into the UIs of applications has a lot of upside with little downside, the author says (HAKINMHAN/Shutterstock)
For instance, the GenAI bot that integrates with the app should already be trained, and it should also be able to train itself over time to adapt to the specific needs of individual users and organizations. Advanced organizations can certainly manage training the GenAI bot if they possess the skills in-house, but the vendors’ training should be sufficient to see plenty of ROI. Likewise, since most applications are delivered on a SaaS basis, running in the cloud, scarce GenAI hardware shouldn’t be a problem.
Hallucination, of course, remains an issue, but only if GenAI is creating content rather than serving as an interface between the user and the application. For instance, if GenAI is incorporated into an ERP or a business intelligence platform, when a user asks for information, the bot isn’t analyzing and retrieving data. Instead, it’s translating a natural language request into a request the platform will understand. The bot then relays this information — which is reliable because it originates from a trusted source — in whatever format the user desires.
GenAI’sROI has the potential to be enormous. GenAI extends access to complex and powerful platforms from upper management all the way out to frontline workers. Sales reps could ask the ERP platform whether a specific product is available in the warehouse, and a retail store manager can ask the BI platform what products are moving the fastest and need to be reordered immediately. These employees won’t need dashboards created for them, and they won’t need to flip through many different pages to find the specific data they need. GenAI takes their request, relays it to the application, and then returns the results to the user in a format that’s easy to understand.
As GenAI matures, other use cases will become feasible for organizations to deploy. But right now, organizations will see the fastest ROI from GenAI that’s embedded into critical SaaS applications to enable organizations to make data-driven decisions from the C-suite to the frontlines and everywhere in between.
About the author: Saurabh Abhyankar has been innovating in the analytics market for 20 years and holds a number of patents in self-service analytics, the semantic graph, and HyperIntelligence. Since 2016, he has held various product leadership positions at MicroStrategy including SVP of Product Management and EVP of Marketing.
Related Items:
LLMs and GenAI: When To Use Them
GenAI Begins Journey Into Trough of Disillusionment
GenAI Adoption: Show Me the Numbers