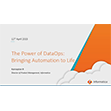
Hitachi Vantara Urges Businesses to Invest in Data Infrastructure to Unlock AI Potential
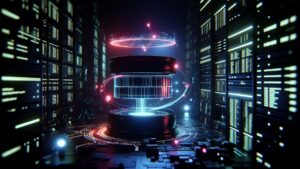
(Shutterstock AI Generator/Shutterstock)
The explosive growth of GenAI has unlocked vast amounts of untapped data within enterprises, presenting both opportunities and challenges for IT leaders. While large language models (LLMs) promise transformative potential, many companies are still struggling to secure returns on their AI investments.
A recent survey from Hitachi Vantara reveals that nearly 37% of U.S. companies consider data quality their biggest challenge when deploying AI projects. Many IT leaders are failing to prioritize effective data management, putting their AI efforts at risk.
According to Hitachi Vantara’s State of Data Infrastructure Global Report 2024, the challenge of ensuring data quality is not going to get any easier. The data solutions provider predicts that the amount of data is set to increase by 122% by 2026. As a result, storing, labeling, and managing data to facilitate AI models is only going to get more challenging.
The report is based on a survey of 1,200 business executives and IT decision-makers from large organizations in 15 countries. It explores the growing demands on data infrastructure as AI evolves and organizations face unprecedented data storage challenges.
The Hitachi Vantara report highlights that IT leaders are inspired by the success of early AI adopters. A recent study commissioned by Google Cloud revealed that 86% of AI early adopters gained an average of 6% in revenue. This trend is reflected in the Hitachi Vantara report, which reveals that more than three-quarters (76%) of respondents consider AI to be a widespread or critical function within their organizations.
Despite the growing reliance on AI, only 38% of respondents report having access to the necessary data when they need it, and just one-third believe the majority of AI model outputs are accurate. Perhaps more concerning is the fact that a staggering 80% of data remains unstructured, creating additional risks as data volumes continue to expand. Many organizations are overlooking ROI analysis or sustainability in their overall AI strategy.
If IT leaders are aware of these issues, what actions are they taking to mitigate the risks? The survey reveals that only a few are taking steps to improve data quality. Only 37% of organizations are focusing on improving the quality of the data used to train AI models and nearly half (47%) don’t tag data for visualization. Even worse, 26% do not check datasets for quality.
“The adoption of AI depends very heavily on the trust of users in the system and in the output. If your early experiences are tainted, it taints your future capabilities,” said Simon Ninan, Senior Vice President of Business Strategy, Hitachi Vantara. “Many people are jumping into AI without a defined strategy or outcome in mind because they don’t want to be left behind, but the success of AI depends on several key factors, including going into projects with clearly defined use cases and ROI targets”.
“It also means investing in modern infrastructure that is better equipped to handle massive data sets in a way that prioritizes data resiliency and energy efficiency. In the long run, infrastructure built without sustainability in mind will likely need rebuilding to adhere to future sustainability regulations.”
The survey shows that the security of data storage is a top priority with 54% of respondents labeling that as their highest area of concern within their organization’s infrastructure. Additionally, 74% agree that any data loss would be catastrophic to business operations, while 73% fear hackers will leverage AI-optimized tools to gain unauthorized access.
Hitachi Vantara emphasizes investing in modern architecture to handle growing data volumes. It recommends focusing on data resilience, sustainability, and security. The survey showed that sustainably currently doesn’t rank as high on the priority list as you would expect with just 32% prioritizing sustainability.
The findings indicate that organizations are focusing on large-scale models. The report suggests prioritizing specialized LLMs that are significantly less energy-hungry and offer greater performance potential. This could also help organizations have a more defined AI strategy.
What can organizations do to get the most value from their data? Hitachi recommends leveraging third-party expertise for critical areas such as data storage, data processing, and AI model creation. This could help create more resilient data capable of supporting long-term growth.
Related Items
Cutting-Edge Infrastructure Best Practices for Enterprise AI Data Pipelines
What We’ve Learned from Over Two Decades of Data Virtualization