2019 – DataOps: A Return to Data Engineering
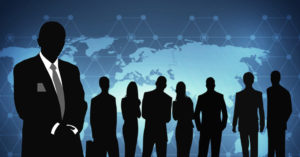
When the big data boom fizzled out around 2016, AI was there to carry the torch forward. But by 2019, the shine was starting to wear off AI. The culprit? Bad data, as usual.
That’s not to say that 2019 marked a return to big data. After all, data volumes have been growing at a geometric rate for the duration of this publication’s existence (since 2011, hence the Decade of Datanami). Data has always been “big.”
But what happened instead that year was a refocus on the centrality and importance of clean, well-managed data to any advanced analytic or AI endeavors. It’s been said before, but it bears repeating: to be data-driven, you must have good data. But good data is hard to find.
The data divide shows up in various ways, from the poor overall state of enterprise data and data lakes turned into data swamps, to dark data hidden from view, and data science professionals spending the majority of their time cleaning data.
It struck us as odd that, in this age of advanced AI, that companies are entirely dependent on the extract, transform, and load (ETL) process to fuel AI projects (and the advent of ELT does not count as progress, since the all-important transformation component is done essentially the same way). In March 2019, we wrote, “Can we stop doing ETL Yet?”The answer? Not if we want to do advanced analytics AI.
We spotted several trends relating to the sorry state of data, and several of them had to do with the personnel that enterprises hire to execute their data strategies. Data engineers continued to be much sought-after, particularly with the occupants of the C suite, while some companies took extended looks at data stewards, the dedicated librarians of our data estates. However, the heavy burden that data folks were bearing was starting to have an impact on worker performance. No matter how big your shovel is, there is always more data to move.
The rapidly maturing world of cloud computing seemed tailor made to at least help us with the data dilemma. After all, the public clouds essentially made big data obsolete by taking all the best parts of Hadoop and repacking them into something that worked. Won’t they help us out of the data mess? The truth is, the rise of clouds just serves to complicate the data investments for most companies. The cloud in the end turns into just another silo.
The data lakehouse, which implements a happy medium between the wet-and-wild data lakes and the buttoned-up data warehouse, began to gain some serious traction, thanks to the folks at Databricks and their Delta Lake offering. This is a trend that has accelerated into 2021.
Data catalogs continued their assault on the consciousness of data professionals everywhere, while data pipeline automation tools like Airflow and Kubeflow grew in popularity. The nascent practice of DataOps, which seeks to automate tasks like data extraction, data cleansing, and data preparation for downstream analytics and AI tasks, also started to grab business leaders’ attention.
At the end of the day, there is no free lunch in big data–there never was, and there never will be. While automation and AI can alleviate some of the data burdens, it scarcely helps to keep up with ever-growing volumes of data. In 2019, we learned, again, that careful planning and execution of the more rudimentary aspects of data management remains critical to success for higher order projects like advanced analytics and AI.
Related Items:
2020 – COVID-19 — Kicking Digital Transformation Into Overdrive
2018 – GDPR and the Big Data Backlash
2017 – AI, Deep Learning, and GPUs
2016 – Clouds, Clouds Everywhere
2015 – Spark Takes the Big Data World by Storm
2013 – The Flourishing Open Source Ecosystem