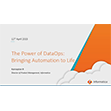
Monte Carlo Survey: Data Engineers Spend Two Days Per Week on Bad Data
SAN FRANCISCO, Aug. 9, 2022 — Monte Carlo, the data reliability company and creator of the data observability category, today announced the initial results of its 2022 data quality survey, which found that data professionals are spending 40% of their time evaluating or checking data quality and that poor data quality impacts 26% of their companies’ revenue.
The report, commissioned by Monte Carlo and conducted by Wakefield Research between April 28 and May 11, 2022, found that 75% of the 300 data professionals surveyed take four or more hours to detect a data quality incident and about half said it takes an average of nine hours to resolve the issue once identified. Worse, 58% said the total number of incidents has increased somewhat or greatly over the past year, often as a result of more complex pipelines, bigger data teams, greater volumes of data, and other factors.
Today, the average organization experiences about 61 data-related incidents per month, each of which takes an average of 13 hours to identify and resolve. This adds up to an average of about 793 hours per month, per company.
However, 61 incidents only represents the number of incidents known to respondents. Proprietary data from the Monte Carlo platform suggests the average organization experiences about 70 data incidents per year for every thousand tables in their environment.
“In the mid-2010s, organizations were shocked to learn that their data scientists were spending about 60% of their time just getting data ready for analysis,” said Barr Moses, Monte Carlo CEO and co-founder. “Now, even with more mature data organizations and advanced stacks, data teams are still wasting 40% of their time troubleshooting data downtime. Not only is this wasting valuable engineering time, but it’s also costing precious revenue and diverting attention away from initiatives that move the needle for the business. These results validate that data reliability is one of the biggest and most urgent problems facing today’s data and analytics leaders. ”
Nearly half of respondent organizations measure data quality most often by the number of customer complaints their company receives, highlighting the ad hoc – and reputation damaging – nature of this important element of modern data strategy.
The Business Cost of Data Downtime
“Garbage in, garbage out” aptly describes the impact data quality has on data analytics and machine learning. If the data is unreliable, so are the insights derived from it.
In fact, on average, respondents said bad data impacts 26% of their revenue. This validates and supplements other industry studies that have uncovered the high cost of bad data. For example, Gartner estimates poor data quality costs organizations an average $12.9 million every year.
Nearly half said business stakeholders are impacted by issues the data team doesn’t catch most of the time, or all the time.
In fact, according to the survey, respondents that conducted at least three different types of data tests for distribution, schema, volume, null or freshness anomalies at least once a week suffered fewer data incidents (46) on average than respondents with a less rigorous testing regime (61). However, testing alone was insufficient and stronger testing did not have a significant correlation with reducing the level of impact on revenue or stakeholders.
“Testing helps reduce data incidents, but no human being is capable of anticipating and writing a test for every way data pipelines can break. And if they could, it wouldn’t be possible to scale across their always changing environment,” said Lior Gavish, Monte Carlo CTO and co-founder. “Machine learning-powered anomaly monitoring and alerting through data observability can help teams close these coverage gaps and save data engineers’ time.”
Within Six Months, 90% of Organizations Will Invest or Plan to Invest in Data Quality
Last year, organizations spent $39.2 billion on cloud databases such as Snowflake, Databricks and Google BigQuery. This year, 88% of respondent organizations are already investing or planning to invest in data quality solutions within six months.
Data observability is one such data quality solution. Leading data teams at organizations like JetBlue, Vimeo, and Affirm leverage automated, end-to-end data observability to detect, resolve and prevent data incidents and lower data downtime at scale. For example, digital advertising software provider Choozle reduced its data downtime by 88% using Monte Carlo.
To read the full report, including commentary and reactions from nearly two dozen data leaders at companies like SoFi, AutoTrader, and PagerDuty, click here.
About Monte Carlo
As businesses increasingly rely on data to power digital products and drive better decision-making, it’s mission-critical that this data is trustworthy and reliable. Monte Carlo, the data reliability company, solves the costly problem of broken data through their fully automated, SOC-2 certified data observability platform. Billed by Forbes as the New Relic for data teams and backed by Accel, Redpoint Ventures, GGV Capital, ICONIQ Growth, Salesforce Ventures, and IVP, Monte Carlo empowers companies to trust their data.
Source: Monte Carlo